Garbage classification method based on hybrid convolutional neural network
A convolutional neural network and garbage classification technology, applied in the field of garbage classification and recycling, can solve the problems of long training time and low garbage classification accuracy, and achieve the effect of improving representation ability, shortening training time, and good fitting.
- Summary
- Abstract
- Description
- Claims
- Application Information
AI Technical Summary
Problems solved by technology
Method used
Image
Examples
specific Embodiment approach 1
[0027] Specific implementation mode one: as figure 1 As shown, a kind of garbage classification method based on hybrid convolutional neural network described in this embodiment comprises the following steps:
[0028] Step 1, load the garbage image, and preprocess the garbage image to obtain the preprocessed garbage image;
[0029] Step 2, building a hybrid convolutional neural network, inputting the preprocessed garbage image obtained in step 1 into the hybrid convolutional neural network for training, to obtain a trained hybrid convolutional neural network;
[0030] The structure of the hybrid convolutional neural network is:
[0031] Starting from the input end of the hybrid convolutional neural network, the hybrid convolutional neural network sequentially includes the first network module, the second network module, the third network module, the fourth network module, the flattening layer, the first fully connected layer, the first The batch normalization layer after the fu...
specific Embodiment approach 2
[0035] Specific embodiment 2: The difference between this embodiment and specific embodiment 1 is that in the first step, the garbage image is preprocessed to obtain the preprocessed garbage image; the specific process is:
[0036] Obtain the preprocessed garbage image by performing data enhancement and normalization processing on the loaded garbage image;
[0037] The data augmentation method includes randomly zooming, flipping, translating and rotating the garbage image.
[0038] Generally speaking, a more successful neural network requires a large number of parameters, and making these parameters work properly requires a large amount of data for training, but in reality there is often not so much data. Considering that the trash image database TrashNet has relatively few samples, data enhancement is performed on the trash image during preprocessing to increase the number of training samples. It can not only increase the amount of training data and improve the generalizatio...
specific Embodiment approach 3
[0039] Specific implementation mode three: the difference between this implementation mode and specific implementation mode one is:
[0040] Batch normalization is added after each convolutional layer and fully connected layer to further enhance the feature extraction ability of the model, which can effectively avoid gradient disappearance and gradient explosion, and reduce the structural complexity of the model.
[0041] The convolutional layer is used to extract image features, and the BN layer is used to improve the generalization ability of the network, disrupt the training data and speed up the convergence speed of the model. During training, BN is calculated based on each small batch. The mean and variance corresponding to each batch of data during training are recorded, and they are used to calculate the mean and variance of the entire training set. The calculation formula is:
[0042]
[0043]
[0044] E[x]←E β [μ β ]
[0045]
[0046] Among them, m refers ...
PUM
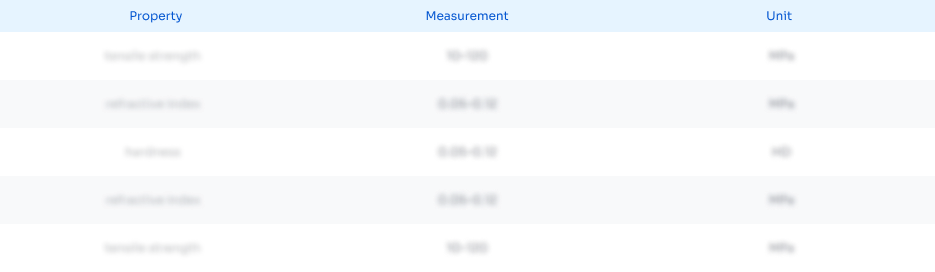
Abstract
Description
Claims
Application Information
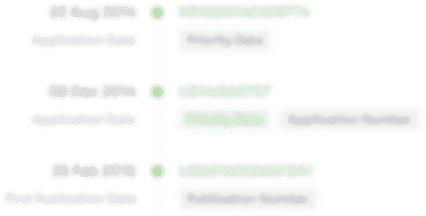
- R&D
- Intellectual Property
- Life Sciences
- Materials
- Tech Scout
- Unparalleled Data Quality
- Higher Quality Content
- 60% Fewer Hallucinations
Browse by: Latest US Patents, China's latest patents, Technical Efficacy Thesaurus, Application Domain, Technology Topic, Popular Technical Reports.
© 2025 PatSnap. All rights reserved.Legal|Privacy policy|Modern Slavery Act Transparency Statement|Sitemap|About US| Contact US: help@patsnap.com