U-shaped cavity full-convolution integral segmentation network identification model based on remote sensing image
A remote sensing image and network recognition technology, applied in biological neural network models, scene recognition, character and pattern recognition, etc., can solve the problems of large scale changes of ground object information, complex structure of ground object information such as overpasses, etc., to achieve accurate extraction, The effect of expanding the receptive field and reducing the training parameters
- Summary
- Abstract
- Description
- Claims
- Application Information
AI Technical Summary
Problems solved by technology
Method used
Image
Examples
Embodiment 1
[0078] Embodiment 1: The present invention is tested by utilizing a scene image of Gaofen No. 2 image. One scene of Gaofen-2 image covers about 506.25 square kilometers, the spatial resolution is 0.8m, the image size is 29200×27200, and the single fused image is about 6G. In addition, the coverage rate of open-pit mines in the single-scene image is low, that is, open-pit Mine is sparse for imagery. Therefore, directly using the original image training will lead to a large machine load rate and a low positive sample ratio (very low effective data ratio). Training based on this unbalanced sample ratio will improve the accuracy of image feature extraction and category discrimination. Therefore, a series of data preprocessing such as labeling, effective area selection, mine area cutting, and data amplification are performed on the fused remote sensing data to obtain a learning data set that can be used for the recognition model, including training sets, verification sets, and test...
Embodiment 2
[0087] Embodiment 2: Test the present invention by using the data of Gaofen No. 2 urban area again. Gaofen No. 2 urban area covers about 506.25 square kilometers, with a spatial resolution of 0.8m, an image resolution of 29200×27200, and a single fused image of about 6G. Therefore, directly using the original image training will lead to a large machine load rate and a low positive sample ratio (very low effective data ratio). Training based on this unbalanced sample ratio will improve the accuracy of image feature extraction and category discrimination. Therefore, the fused remote sensing is subjected to a series of data preprocessing such as label cutting and data amplification. Before that, a series of processing such as atmospheric correction, orthorectification, image registration, and image fusion must be performed. Here, we preprocess the recognition algorithm based on some pre-fusion preprocessing operations and images obtained after fusion. The original images of TIF ...
PUM
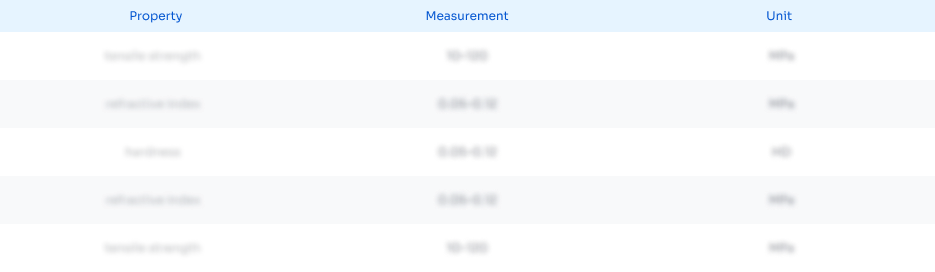
Abstract
Description
Claims
Application Information
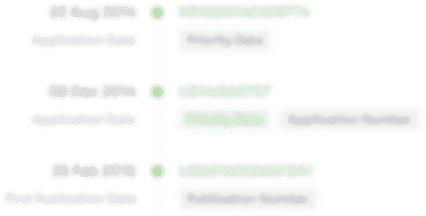
- Generate Ideas
- Intellectual Property
- Life Sciences
- Materials
- Tech Scout
- Unparalleled Data Quality
- Higher Quality Content
- 60% Fewer Hallucinations
Browse by: Latest US Patents, China's latest patents, Technical Efficacy Thesaurus, Application Domain, Technology Topic, Popular Technical Reports.
© 2025 PatSnap. All rights reserved.Legal|Privacy policy|Modern Slavery Act Transparency Statement|Sitemap|About US| Contact US: help@patsnap.com