Label noise detection method based on multi-granularity relative density
A relative density and noise detection technology, applied in the direction of instruments, character and pattern recognition, computer components, etc., can solve the problems of not making full use of the noise contrast characteristics of labels, poor information quality, and not much available information, etc., to reduce The risk of overfitting, the effect of reducing time overhead and good generalization ability
- Summary
- Abstract
- Description
- Claims
- Application Information
AI Technical Summary
Problems solved by technology
Method used
Image
Examples
Embodiment Construction
[0029] The present invention is a label noise detection method based on multi-granularity relative density. How to deal with label noise has become a research hotspot in the field of machine learning, covering many practical application areas. The current mainstream research methods are divided into noise accommodation and noise filtering. When the training data is polluted by label noise, an obvious solution is to clean the training data yourself, similar to outlier or anomaly detection. This scheme belongs to noise filtering.
[0030] S1 divides the data set into K clusters, and calculates the relative density of improvement in granularity for each sample. The improved relative density is defined as first calculating the centroids of the positive and negative samples respectively, and then calculating the distances from each sample to the centroid of the same kind and the centroid of the different kind, and using the ratio of the distances as the improved relative density a...
PUM
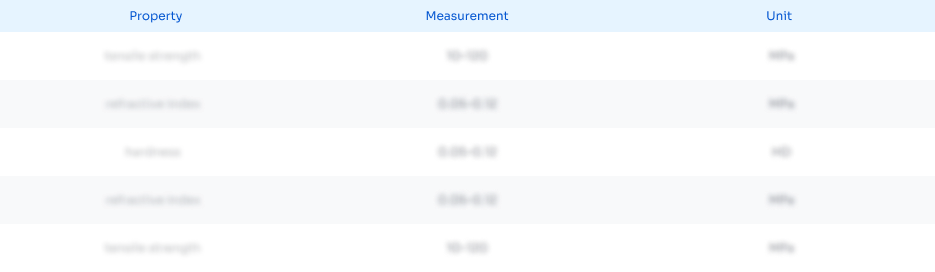
Abstract
Description
Claims
Application Information
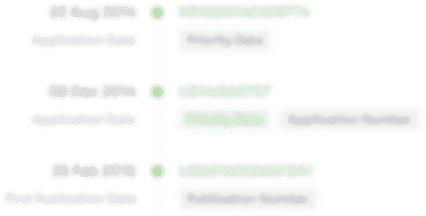
- R&D Engineer
- R&D Manager
- IP Professional
- Industry Leading Data Capabilities
- Powerful AI technology
- Patent DNA Extraction
Browse by: Latest US Patents, China's latest patents, Technical Efficacy Thesaurus, Application Domain, Technology Topic, Popular Technical Reports.
© 2024 PatSnap. All rights reserved.Legal|Privacy policy|Modern Slavery Act Transparency Statement|Sitemap|About US| Contact US: help@patsnap.com