Rectal cancer pathology image classification method based on multi-channel collaborative capsule network
A pathology, multi-channel technology, applied in the direction of understanding medical/anatomical models, instruments, calculations, etc., can solve problems such as no rotation, loss of spatial information, etc., to improve generalization ability and overcome the effect of loss of spatial information
- Summary
- Abstract
- Description
- Claims
- Application Information
AI Technical Summary
Problems solved by technology
Method used
Image
Examples
Embodiment Construction
[0079] The present invention will be described in detail below with reference to specific embodiments, but the protection scope of the present invention is not limited to the following embodiments.
[0080] like figure 1 As shown, the main structure of the system in this implementation case includes: an image data acquisition module, an image data preprocessing module, an image feature extraction module, a network training module, and a test evaluation module; the image data acquisition module is used for crawling and collecting colon cancer. Pathological images and structured data processing; image data preprocessing module includes using Min-Max Normalization to normalize data, using One-Hot Encoder to label image categories, and using data augmentation technology to enhance data robustness properties and generalization; image feature extraction module, using weight self-adjusting feature fusion technology to extract image features; network training module, using capsule netw...
PUM
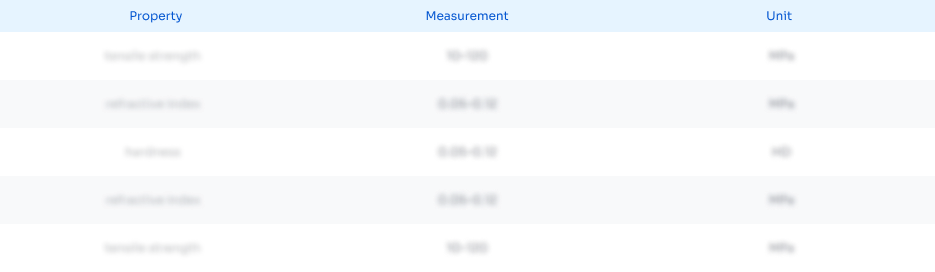
Abstract
Description
Claims
Application Information
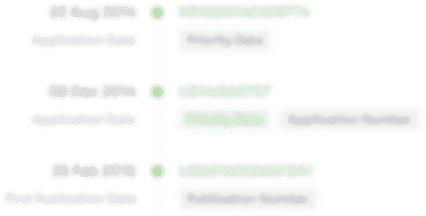
- R&D Engineer
- R&D Manager
- IP Professional
- Industry Leading Data Capabilities
- Powerful AI technology
- Patent DNA Extraction
Browse by: Latest US Patents, China's latest patents, Technical Efficacy Thesaurus, Application Domain, Technology Topic.
© 2024 PatSnap. All rights reserved.Legal|Privacy policy|Modern Slavery Act Transparency Statement|Sitemap