Chaotic time sequence prediction method based on particle swarm optimization and auto-encoder
A chaotic time series, particle swarm optimization technology, applied in forecasting, neural learning methods, biological neural network models, etc., can solve problems such as air pollution
- Summary
- Abstract
- Description
- Claims
- Application Information
AI Technical Summary
Problems solved by technology
Method used
Image
Examples
specific Embodiment
[0102] Taking the time series of Air Quality Index (AQI) in Beijing as the research object, the data comes from the UCI machine learning database.
[0103] The data interval is from 0:00 on January 1, 2010 to 23:59 on December 31, 2014, a total of 43800 groups of samples, each group of samples includes 5-dimensional variables, which are PM collected every hour 2.5 Concentration, dew point temperature, air temperature, air pressure and cumulative wind speed.
[0104] The first 75% of the data (32850 samples, from 0:00 on January 1, 2010 to 17:59 on September 1, 2013) were used as training samples for this method, and the output weights of the feed-forward neural network were optimized with the MPSO algorithm.
[0105] Such as image 3 As shown in , the trained MPSO-SAE is simulated (at this time, the feedforward neural network has obtained the optimal output weight), and the fitting situation between the simulated value and the sample is compared to test the generalization abili...
PUM
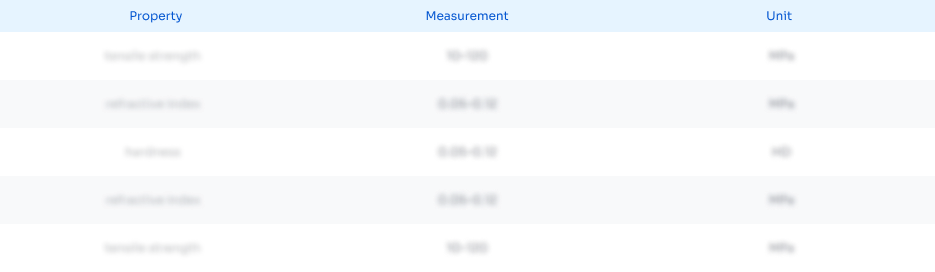
Abstract
Description
Claims
Application Information
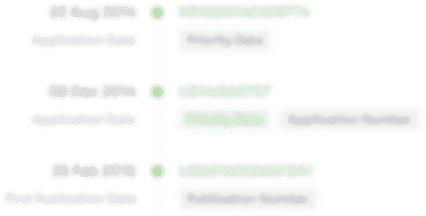
- R&D Engineer
- R&D Manager
- IP Professional
- Industry Leading Data Capabilities
- Powerful AI technology
- Patent DNA Extraction
Browse by: Latest US Patents, China's latest patents, Technical Efficacy Thesaurus, Application Domain, Technology Topic, Popular Technical Reports.
© 2024 PatSnap. All rights reserved.Legal|Privacy policy|Modern Slavery Act Transparency Statement|Sitemap|About US| Contact US: help@patsnap.com