Vehicle detection method with granularity-level multi-scale characteristic based on asymmetric convolution
A vehicle detection, asymmetric technology, applied in the direction of neural learning methods, instruments, biological neural network models, etc., can solve the problems of low real-time performance and robustness, poor detection performance of multi-scale problems, etc.
- Summary
- Abstract
- Description
- Claims
- Application Information
AI Technical Summary
Problems solved by technology
Method used
Image
Examples
Embodiment Construction
[0073] The technical solutions in the embodiments of the present invention will be clearly and completely described and discussed below in conjunction with the accompanying drawings of the present invention. Obviously, what is described here is only a part of the examples of the present invention, not all examples. Based on the present invention All other embodiments obtained by persons of ordinary skill in the art without creative efforts fall within the protection scope of the present invention.
[0074] Such as figure 1 As shown, this embodiment discloses a vehicle detection method with granularity-level multi-scale characteristics based on asymmetric convolution, including the following steps:
[0075] Step S1: Obtain a number of pictures containing vehicles through the video stream, and make a vehicle target detection data set, including a training set and a test set;
[0076] Step S2: Design an asymmetric convolution AC unit;
[0077] Further, the design of the asymmet...
PUM
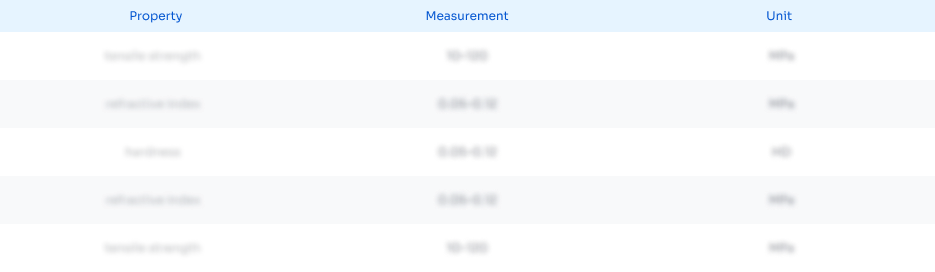
Abstract
Description
Claims
Application Information
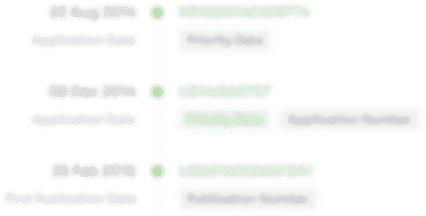
- R&D
- Intellectual Property
- Life Sciences
- Materials
- Tech Scout
- Unparalleled Data Quality
- Higher Quality Content
- 60% Fewer Hallucinations
Browse by: Latest US Patents, China's latest patents, Technical Efficacy Thesaurus, Application Domain, Technology Topic, Popular Technical Reports.
© 2025 PatSnap. All rights reserved.Legal|Privacy policy|Modern Slavery Act Transparency Statement|Sitemap|About US| Contact US: help@patsnap.com