Aramid paper honeycomb gluing defect detection method
A technology of defect detection and aramid paper, applied in the field of visual inspection, can solve problems such as no uniform pattern, poor imaging, waste of raw materials, etc., and achieve the effect of increasing the number of samples, reducing pressure, and strong generalization ability
- Summary
- Abstract
- Description
- Claims
- Application Information
AI Technical Summary
Problems solved by technology
Method used
Image
Examples
Embodiment Construction
[0020] Specific embodiments of the present invention will be discussed in detail below in conjunction with the accompanying drawings.
[0021] combine figure 2 , image 3 As shown in the decoder and encoder, the present invention provides a method for detecting aramid paper honeycomb gluing defects, including aramid paper, on which there are defects generated in the gluing process; industrial cameras, used for The defect image information of aramid paper is obtained; the depth feature encoder is used to fully encode the defect image information of different types and shapes; the feature decoder is used to accurately locate the encoded defect image information; the detection steps are as follows: figure 1 detection protocol workflow,
[0022] The first step is to perform image enhancement processing in different ways on the defect image information obtained by the industrial camera to obtain different defect image samples;
[0023] In the second step, the deep feature encod...
PUM
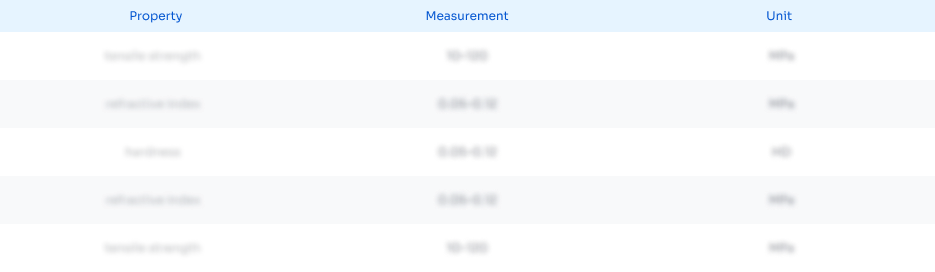
Abstract
Description
Claims
Application Information
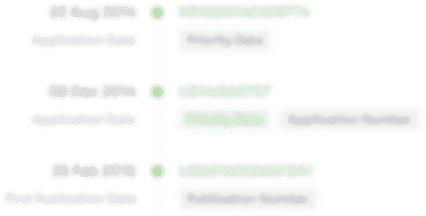
- R&D
- Intellectual Property
- Life Sciences
- Materials
- Tech Scout
- Unparalleled Data Quality
- Higher Quality Content
- 60% Fewer Hallucinations
Browse by: Latest US Patents, China's latest patents, Technical Efficacy Thesaurus, Application Domain, Technology Topic, Popular Technical Reports.
© 2025 PatSnap. All rights reserved.Legal|Privacy policy|Modern Slavery Act Transparency Statement|Sitemap|About US| Contact US: help@patsnap.com