Target region extraction method for multi-modal medical image based on convolutional neural network
A technology of convolutional neural network and target area, which is applied in the field of target area extraction of multimodal medical images, can solve the problems of underutilization, high manpower consumption, underutilization of MRI differences of different parameters, etc., to overcome time-consuming Efforts to increase effort, improve accuracy, and overcome problems of subjective variability
- Summary
- Abstract
- Description
- Claims
- Application Information
AI Technical Summary
Problems solved by technology
Method used
Image
Examples
Embodiment Construction
[0043] The present invention will be further described in detail below in conjunction with the embodiments, so that those skilled in the art can implement it with reference to the description.
[0044] It should be understood that terms such as "having", "comprising" and "including" used herein do not exclude the presence or addition of one or more other elements or combinations thereof.
[0045] A method for extracting a target region of a multimodal medical image based on a convolutional neural network in this embodiment includes the following steps:
[0046] 1) Construct a mask region convolutional neural network for target region extraction in multimodal medical images:
[0047] refer to figure 1 , the mask area convolutional neural network includes a multi-modal medical image feature extraction network, a target region proposal network, and a head network, and the multi-modal medical image feature extraction network extracts multi-level fusion feature maps of multi-modal...
PUM
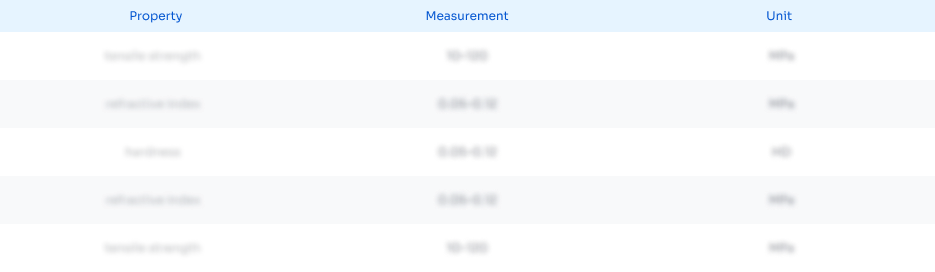
Abstract
Description
Claims
Application Information
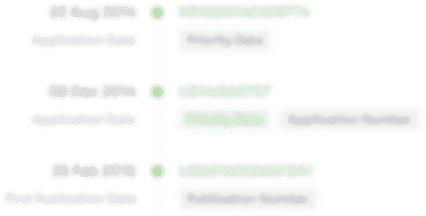
- R&D Engineer
- R&D Manager
- IP Professional
- Industry Leading Data Capabilities
- Powerful AI technology
- Patent DNA Extraction
Browse by: Latest US Patents, China's latest patents, Technical Efficacy Thesaurus, Application Domain, Technology Topic.
© 2024 PatSnap. All rights reserved.Legal|Privacy policy|Modern Slavery Act Transparency Statement|Sitemap