Skew time series anomaly detection method based on cost-sensitive hybrid network
A cost-sensitive, time-series technology, applied in neural learning methods, biological neural network models, instruments, etc., can solve problems such as insufficient learning of minority sample features, low detection accuracy, and decreased classification accuracy
- Summary
- Abstract
- Description
- Claims
- Application Information
AI Technical Summary
Problems solved by technology
Method used
Image
Examples
Embodiment Construction
[0104] The present invention will be described in detail below in conjunction with the accompanying drawings and specific embodiments.
[0105] Such as figure 1 and 2 As shown, the present invention is based on a skewed time series anomaly detection method based on a cost-sensitive hybrid network. First, establish and train a deep convolutional neural network DCNN, a gated recurrent network GRU containing 128 cell units, and a cost-sensitive loss function. A cost-sensitive hybrid network model, in which the local features of the time series are learned through the deep convolutional neural network DCNN, and the sequence features of the time series are learned through the gated recurrent network GRU, and then these features are combined and passed through the Soft- The max classifier is used to classify, and the cost-sensitive loss function is used to measure the similarity between the output result and the real value during the model training process, and then the parameters ...
PUM
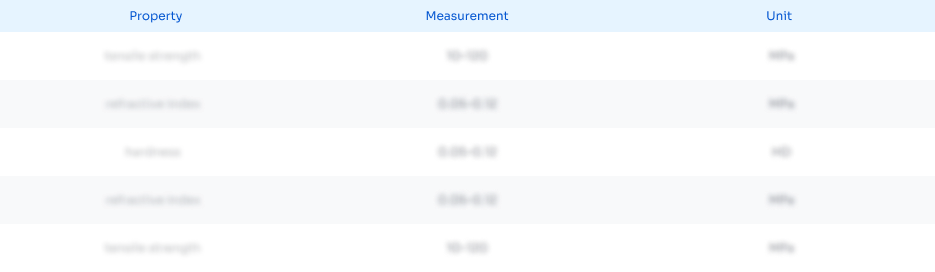
Abstract
Description
Claims
Application Information
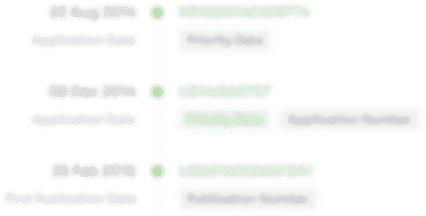
- Generate Ideas
- Intellectual Property
- Life Sciences
- Materials
- Tech Scout
- Unparalleled Data Quality
- Higher Quality Content
- 60% Fewer Hallucinations
Browse by: Latest US Patents, China's latest patents, Technical Efficacy Thesaurus, Application Domain, Technology Topic, Popular Technical Reports.
© 2025 PatSnap. All rights reserved.Legal|Privacy policy|Modern Slavery Act Transparency Statement|Sitemap|About US| Contact US: help@patsnap.com