Foundation meteorological cloud picture classification method based on cross validation deep CNN feature integration
A technology of cross-validation and classification method, which is applied in the field of ground-based meteorological cloud image classification based on cross-validation deep CNN feature integration, can solve the problems of complex calculation of cloud recognition process, and unrobust results of single CNN feature cloud classification. Achieve efficient and robust adaptive cloud-like automatic classification, avoid image preprocessing, and reduce computational complexity
- Summary
- Abstract
- Description
- Claims
- Application Information
AI Technical Summary
Problems solved by technology
Method used
Image
Examples
Embodiment 1
[0038] The ground-based meteorological cloud image classification method based on cross-validation deep CNN feature integration in this embodiment first utilizes a single convolutional neural network model to extract the deep CNN features of ground-based meteorological cloud images, and then performs multiple resampling of CNN features based on cross-validation, Finally, based on the voting strategy of multiple cross-validation and resampling results, the ground-based cloud map cloud shape is identified. Before introducing the specific scheme, some basic concepts and operations are first introduced.
[0039] Data set: record a data set containing n ground-based meteorological cloud images as D n , then D n ={z i ,i=1,...,n}. Among them, z i is the data set D n The i-th cloud image in ;
[0040] Metric Set: Image Dataset D n The index set for each z i The set composed of the subscripts of is denoted as I={1,2,...,n};
[0041] Convolution: Through a convolution kernel, m...
PUM
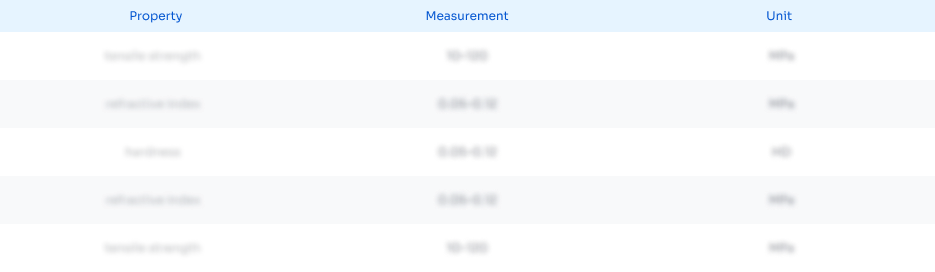
Abstract
Description
Claims
Application Information
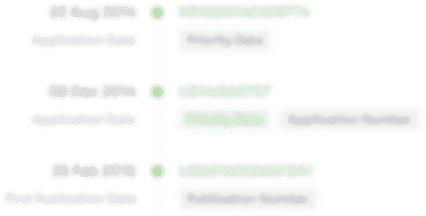
- R&D Engineer
- R&D Manager
- IP Professional
- Industry Leading Data Capabilities
- Powerful AI technology
- Patent DNA Extraction
Browse by: Latest US Patents, China's latest patents, Technical Efficacy Thesaurus, Application Domain, Technology Topic, Popular Technical Reports.
© 2024 PatSnap. All rights reserved.Legal|Privacy policy|Modern Slavery Act Transparency Statement|Sitemap|About US| Contact US: help@patsnap.com