Timing sequence deep survival analysis system with active learning
A technology of survival analysis and active learning, applied in the medical field, can solve problems such as limited model application, right censoring of survival data, poor performance, etc., to achieve the effect of improving performance, expanding the scope of application, and increasing extraction and utilization
- Summary
- Abstract
- Description
- Claims
- Application Information
AI Technical Summary
Problems solved by technology
Method used
Image
Examples
Embodiment Construction
[0037] The present invention will be described in further detail below in conjunction with the accompanying drawings and specific embodiments.
[0038] Censored data in survival analysis are usually divided into three cases: left censored, right censored and interval censored:
[0039] Left censored (Left Censored): It means that the occurrence time of the final event can only be determined before a certain point in time.
[0040] Right Censored: It means that the occurrence time of the final event can only be determined after a certain point in time.
[0041] Interval censored (Interval Censored): It means that the occurrence time of the final event can be determined within a certain time interval.
[0042] Non-censored data (Failure Data) means that the time point at which the final event occurred was accurately observed, and complete survival data were obtained.
[0043] The Cox regression model, also known as the proportional hazards model, analyzes the relationship betw...
PUM
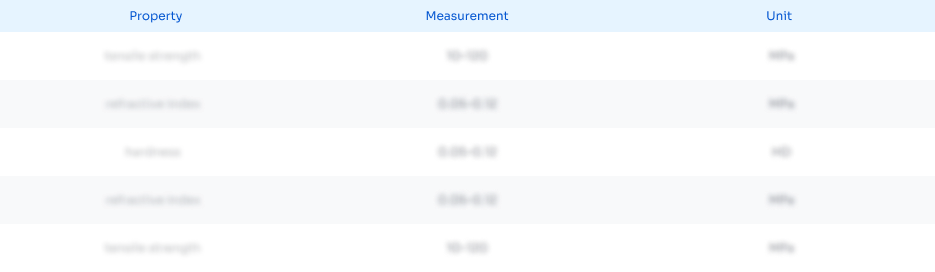
Abstract
Description
Claims
Application Information
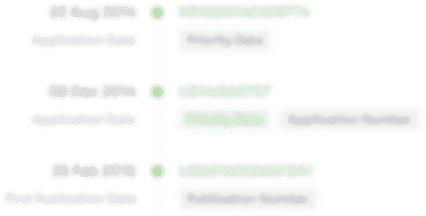
- R&D
- Intellectual Property
- Life Sciences
- Materials
- Tech Scout
- Unparalleled Data Quality
- Higher Quality Content
- 60% Fewer Hallucinations
Browse by: Latest US Patents, China's latest patents, Technical Efficacy Thesaurus, Application Domain, Technology Topic, Popular Technical Reports.
© 2025 PatSnap. All rights reserved.Legal|Privacy policy|Modern Slavery Act Transparency Statement|Sitemap|About US| Contact US: help@patsnap.com