Viewpoint adjustment-based graph convolution cycle network skeleton action recognition method and system
A technology of action recognition and recurrent neural network, which is applied in the field of skeletal action recognition of graph convolutional recurrent network based on viewpoint adjustment, can solve the problems of different observation angles, different recognition results, and low accuracy of action recognition, achieving broad application prospects, The effect of improving accuracy
- Summary
- Abstract
- Description
- Claims
- Application Information
AI Technical Summary
Problems solved by technology
Method used
Image
Examples
Embodiment 1
[0029] Such as figure 1 As shown, Embodiment 1 of the present disclosure provides a skeletal action recognition method based on a viewpoint adjustment based on a graph convolutional loop network, including the following steps:
[0030] (1) Preprocess the acquired action data and use the NTU-RGB+D dataset as the action recognition dataset. This dataset is the largest action data and provides 3D bone coordinates, including 60 different actions, including The two benchmarks of cross-perspective and cross-subject;
[0031] Specifically:
[0032] (1-1) Obtain the original body data from the skeleton sequence; obtain the original body data from the skeleton sequence, each body data is a dictionary, including keywords such as the original 3D joint, the original 2D color position, and the frame index of the subject;
[0033] (1-2) Obtain denoising data from the original skeleton sequence; obtain denoising data (joint positions and color positions) from the original skeleton sequence...
Embodiment 2
[0055] Embodiment 2 of the present disclosure provides a skeletal action recognition system based on viewpoint adjustment based on graph convolutional loop network, including:
[0056] The preprocessing module is configured to: preprocess the acquired action data;
[0057] The bone data prediction module is configured to: use the trained graph convolutional cyclic neural network and use the preprocessed data as input to obtain the spatiotemporal information of the bone data;
[0058] The classification module is configured to: use the Softmax function and take the obtained spatio-temporal information as input to obtain a classification result of the skeletal motion.
[0059] The specific identification method is the same as that in Embodiment 1, and will not be repeated here.
Embodiment 3
[0061] Embodiment 3 of the present disclosure provides a medium on which a program is stored, and when the program is executed by a processor, the steps in the viewpoint-adjusted graph convolutional loop network skeleton action recognition method based on viewpoint adjustment as described in Embodiment 1 of the present disclosure are implemented. .
PUM
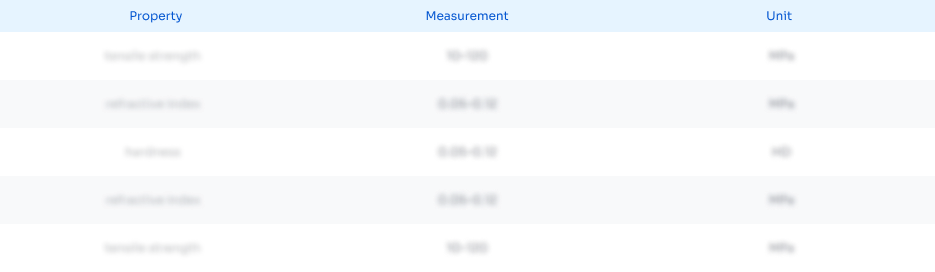
Abstract
Description
Claims
Application Information
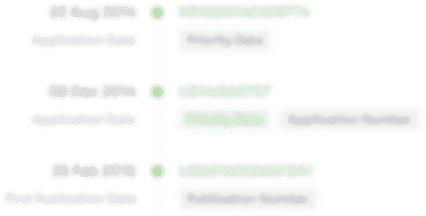
- R&D
- Intellectual Property
- Life Sciences
- Materials
- Tech Scout
- Unparalleled Data Quality
- Higher Quality Content
- 60% Fewer Hallucinations
Browse by: Latest US Patents, China's latest patents, Technical Efficacy Thesaurus, Application Domain, Technology Topic, Popular Technical Reports.
© 2025 PatSnap. All rights reserved.Legal|Privacy policy|Modern Slavery Act Transparency Statement|Sitemap|About US| Contact US: help@patsnap.com