Urinary calculus detection and classification method based on deep learning and imaging omics
A deep learning and radiomics technology, applied in the field of image processing, can solve the problems of poor algorithm robustness, different sources of CT image equipment, and inability to achieve automatic identification and extraction, so as to overcome errors and improve precision and accuracy. Effect
- Summary
- Abstract
- Description
- Claims
- Application Information
AI Technical Summary
Problems solved by technology
Method used
Image
Examples
Embodiment
[0094] The following experiments and evaluation analysis are carried out on the urinary stone detection and classification method based on deep learning and radiomics of the present invention.
[0095] 1. Image acquisition: Obtain routine CT images of urinary calculi with the gold standard.
[0096] 2. On the self-owned labeling system, professional urologists mark the position of the stones on the involved CT images, and label the marked stones according to the gold standard file corresponding to the images. The stones involved in the experiment The types of stones can be divided into two types: calcium stones (mainly calcium oxalate stones) and uric acid stones.
[0097] 3. Divide the data set: the obtained data set is randomly divided into training set, verification set and test set according to the ratio of 6:2:2, wherein the training set and verification set are used to train the stone detection and classification model, and the test set is used for testing performance o...
PUM
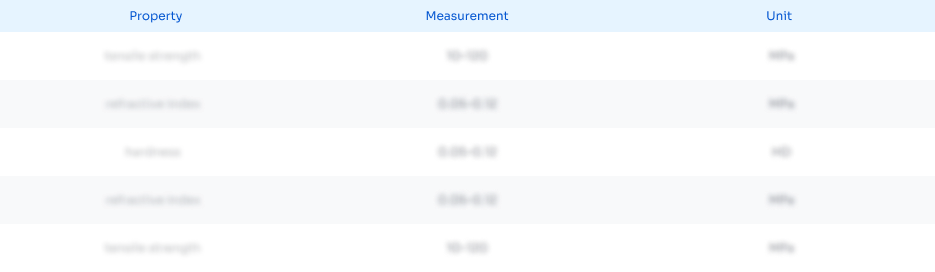
Abstract
Description
Claims
Application Information
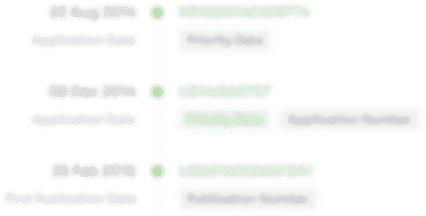
- Generate Ideas
- Intellectual Property
- Life Sciences
- Materials
- Tech Scout
- Unparalleled Data Quality
- Higher Quality Content
- 60% Fewer Hallucinations
Browse by: Latest US Patents, China's latest patents, Technical Efficacy Thesaurus, Application Domain, Technology Topic, Popular Technical Reports.
© 2025 PatSnap. All rights reserved.Legal|Privacy policy|Modern Slavery Act Transparency Statement|Sitemap|About US| Contact US: help@patsnap.com