Small-sample hyperspectral image classification method based on pseudo-label learning
A hyperspectral image, small sample technology, applied in the field of small sample hyperspectral image classification based on pseudo-label learning, can solve problems such as inability to test data generalization, model overfitting, and difficulty in obtaining, to prevent overfitting, The effect of improving the accuracy and improving the classification accuracy
- Summary
- Abstract
- Description
- Claims
- Application Information
AI Technical Summary
Problems solved by technology
Method used
Image
Examples
Embodiment Construction
[0011] The present invention will be further described below in conjunction with the examples, and the present invention includes but not limited to the following examples.
[0012] The present invention provides a small-sample hyperspectral image classification method based on pseudo-label learning, and its basic implementation process is as follows:
[0013] 1. Build a data set
[0014] Take the hyperspectral pixel as the center to sample the surrounding pixels to generate a hyperspectral cube (ie sample), and use the labeled samples to form a small sample data set T, that is, T={(x 1 ,y 1 ),(x 2 ,y 2 ),…,(x n ,y n )},in, Indicates the i-th labeled sample (ie labeled sample), w is the size of the sampling window (can be 3, 5, 7, etc.), d is the number of bands of the hyperspectral image; is the label of the i-th sample, label y i In the form of one-hot encoding, it is an L-dimensional vector, where L is the number of categories; i=1,2,...,n, n is the number of la...
PUM
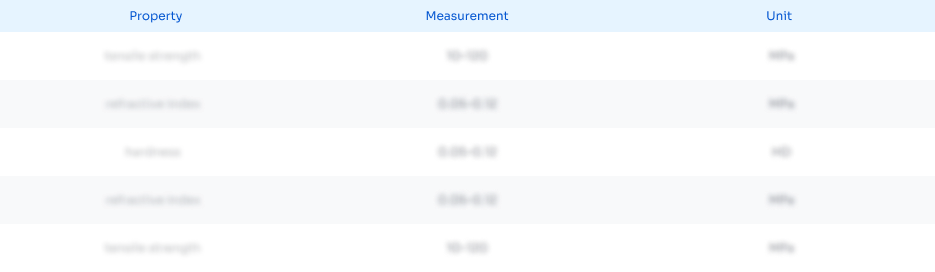
Abstract
Description
Claims
Application Information
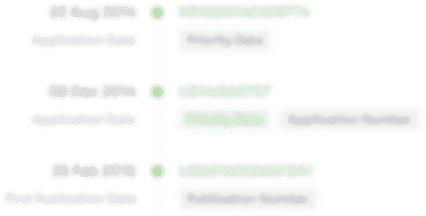
- R&D Engineer
- R&D Manager
- IP Professional
- Industry Leading Data Capabilities
- Powerful AI technology
- Patent DNA Extraction
Browse by: Latest US Patents, China's latest patents, Technical Efficacy Thesaurus, Application Domain, Technology Topic, Popular Technical Reports.
© 2024 PatSnap. All rights reserved.Legal|Privacy policy|Modern Slavery Act Transparency Statement|Sitemap|About US| Contact US: help@patsnap.com