Image super-resolution reconstruction method of supervised convolutional neural network based on multi-scale feature extraction fusion
A convolutional neural network and super-resolution reconstruction technology, applied in the field of image super-resolution reconstruction of supervised convolutional neural networks, can solve problems affecting model reconstruction performance, limitations of model reconstruction performance, poor model reconstruction performance, etc. Solve the problem of gradient disappearance, alleviate the phenomenon of gradient disappearance, and realize the effect of multi-channel propagation
- Summary
- Abstract
- Description
- Claims
- Application Information
AI Technical Summary
Problems solved by technology
Method used
Image
Examples
Embodiment Construction
[0062] In order to enable those skilled in the art to better understand the technical solution of the present invention, the present invention will be described in detail below in conjunction with the accompanying drawings and specific embodiments.
[0063] The embodiment of the present invention discloses an image super-resolution reconstruction method based on multi-scale feature extraction and fusion supervised convolutional neural network, as shown in the figure, which includes the following steps: image preprocessing, image feature extraction and image reconstruction.
[0064] (1) Image preprocessing
[0065] In order to better use the deep learning framework Caffe for training, this preprocessing process is not included in the training network. The image preprocessing part mainly includes the following steps:
[0066] S1: Convert the high-resolution RGB images in the training set to YCbCr color space, and then extract the Y channel,
[0067]
[0068] Y H =Y=0.299R+...
PUM
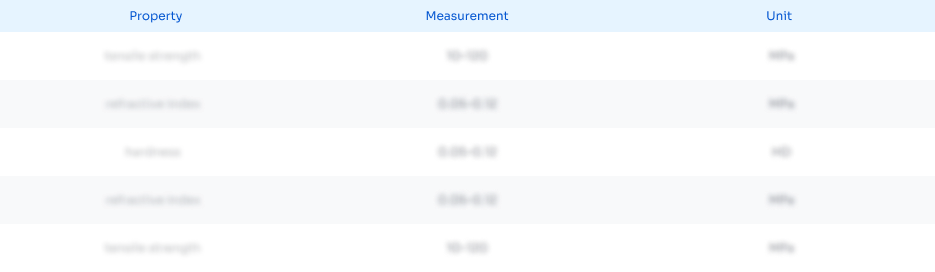
Abstract
Description
Claims
Application Information
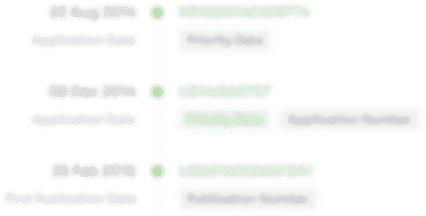
- R&D Engineer
- R&D Manager
- IP Professional
- Industry Leading Data Capabilities
- Powerful AI technology
- Patent DNA Extraction
Browse by: Latest US Patents, China's latest patents, Technical Efficacy Thesaurus, Application Domain, Technology Topic, Popular Technical Reports.
© 2024 PatSnap. All rights reserved.Legal|Privacy policy|Modern Slavery Act Transparency Statement|Sitemap|About US| Contact US: help@patsnap.com