Remote sensing image classification method based on active learning and convolutional neural network
A convolutional neural network and active learning technology, applied in the field of hyperspectral remote sensing image classification, can solve the problems of expensive, time-consuming and labor-intensive label samples, and a large number of other problems
- Summary
- Abstract
- Description
- Claims
- Application Information
AI Technical Summary
Problems solved by technology
Method used
Image
Examples
Embodiment Construction
[0021] The technical solutions in the embodiments of the present invention will be described clearly and in detail below with reference to the drawings in the embodiments of the present invention. The described embodiments are only some of the embodiments of the invention.
[0022] see figure 1 , the concrete steps of the present invention are:
[0023] (1) Obtain hyperspectral remote sensing data according to requirements;
[0024] (2) Perform principal component analysis on hyperspectral remote sensing data and process it into data blocks;
[0025] (3) Divide the data into training set, unlabeled sample set, verification set and test set according to a certain ratio;
[0026] (4) Input the training samples into the convolutional neural network for training, and predict the category of the samples in the unlabeled sample set;
[0027] (5) Use active learning to evaluate the samples in the unlabeled sample set, and sort the confidence of the samples, and select samples wit...
PUM
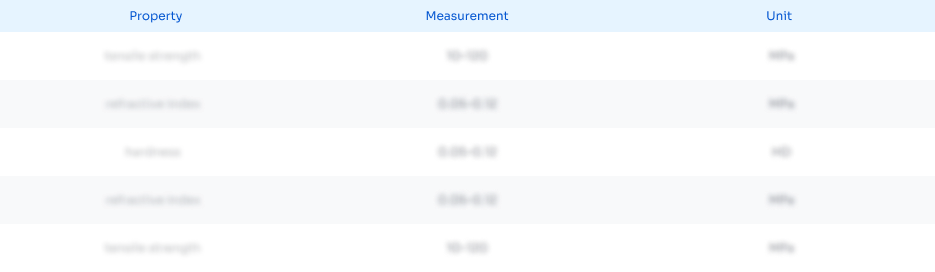
Abstract
Description
Claims
Application Information
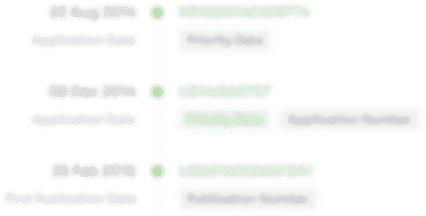
- R&D Engineer
- R&D Manager
- IP Professional
- Industry Leading Data Capabilities
- Powerful AI technology
- Patent DNA Extraction
Browse by: Latest US Patents, China's latest patents, Technical Efficacy Thesaurus, Application Domain, Technology Topic, Popular Technical Reports.
© 2024 PatSnap. All rights reserved.Legal|Privacy policy|Modern Slavery Act Transparency Statement|Sitemap|About US| Contact US: help@patsnap.com