Method for predicting concentration of dissolved gas in transformer oil based on EMD-RF
A technology of transformer oil and dissolved gas, which is applied in neural learning methods, instruments, biological neural network models, etc., and can solve problems such as low prediction accuracy
- Summary
- Abstract
- Description
- Claims
- Application Information
AI Technical Summary
Problems solved by technology
Method used
Image
Examples
Embodiment
[0079] In the present invention, related terms are described as follows:
[0080] Empirical Mode Decomposition Method EMD
[0081] The backpropagation neural network prediction model is abbreviated as: BPNN
[0082] The backpropagation neural network prediction model based on empirical mode decomposition is abbreviated as: EMD-BPNN
[0083] The support vector machine prediction model is abbreviated as: SVM
[0084] The support vector machine prediction model based on empirical mode decomposition is abbreviated as: EMD-SVM
[0085] The random forest prediction model is abbreviated as: RF
[0086] The random forest prediction model based on empirical mode decomposition is abbreviated as: EMD-RF
[0087] In actual operation, the concentration of dissolved gas in transformer oil is affected by oil temperature, oil pressure and operating environment, and its trend is non-stationary fluctuations observed through online monitoring devices. Directly using the random forest model ...
PUM
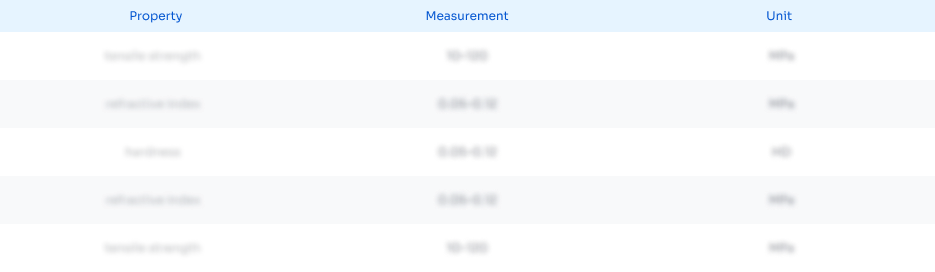
Abstract
Description
Claims
Application Information
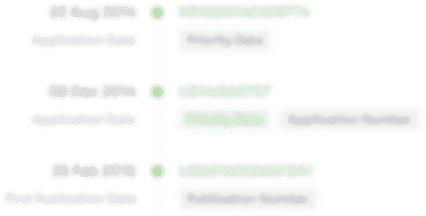
- R&D Engineer
- R&D Manager
- IP Professional
- Industry Leading Data Capabilities
- Powerful AI technology
- Patent DNA Extraction
Browse by: Latest US Patents, China's latest patents, Technical Efficacy Thesaurus, Application Domain, Technology Topic, Popular Technical Reports.
© 2024 PatSnap. All rights reserved.Legal|Privacy policy|Modern Slavery Act Transparency Statement|Sitemap|About US| Contact US: help@patsnap.com