Indoor scene semantic segmentation method based on convolutional neural network
A convolutional neural network and semantic segmentation technology, applied in the field of deep learning semantic segmentation, can solve problems such as multiple storage and computing resources, consumption, and difficult network structure.
- Summary
- Abstract
- Description
- Claims
- Application Information
AI Technical Summary
Problems solved by technology
Method used
Image
Examples
Embodiment Construction
[0058] The present invention will be further described in detail below in conjunction with the accompanying drawings and embodiments.
[0059] Embodiments of the present invention and its implementation process are as follows, and its overall realization block diagram is as follows figure 1 As shown, it includes two processes of training phase and testing phase;
[0060] The specific steps of the described training phase process are:
[0061] Step 1_1: Select Q original indoor scene color images and depth images and real semantic segmentation images corresponding to each original indoor scene image, and form a training set, and record the qth original indoor scene color image in the training set as The depth image is marked as Record its corresponding real semantic segmentation image as Then, the existing one-hot encoding technology (one-hot) is used to process the real semantic segmentation images corresponding to each original indoor scene image in the training set int...
PUM
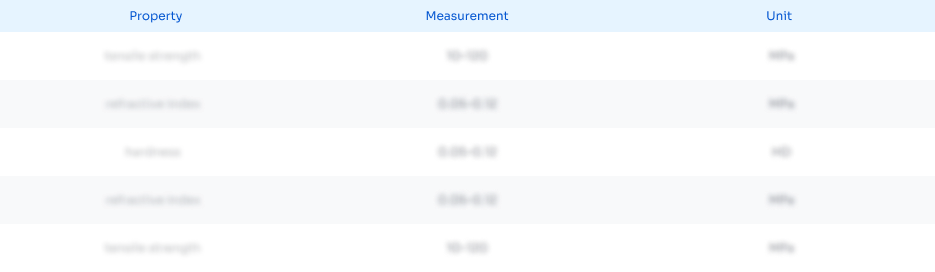
Abstract
Description
Claims
Application Information
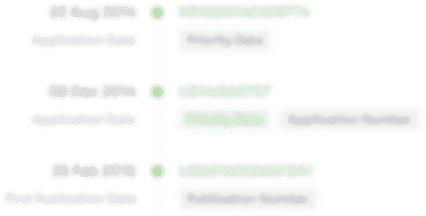
- R&D
- Intellectual Property
- Life Sciences
- Materials
- Tech Scout
- Unparalleled Data Quality
- Higher Quality Content
- 60% Fewer Hallucinations
Browse by: Latest US Patents, China's latest patents, Technical Efficacy Thesaurus, Application Domain, Technology Topic, Popular Technical Reports.
© 2025 PatSnap. All rights reserved.Legal|Privacy policy|Modern Slavery Act Transparency Statement|Sitemap|About US| Contact US: help@patsnap.com