Reinforced learning knowledge graph reasoning method and device based on graph convolutional neural network
A technology of convolutional neural network and knowledge map, applied in the field of reinforcement learning knowledge map reasoning based on graph convolutional neural network, reinforcement learning and graph convolutional neural network technology, can solve the problem of noise introduced by intelligent agent decision-making, and the quality of reasoning path Variation, failure to achieve decision-making strategies and other issues, to achieve the effect of improving decision-making ability, improving decision-making level, and enriching perception
- Summary
- Abstract
- Description
- Claims
- Application Information
AI Technical Summary
Problems solved by technology
Method used
Image
Examples
Embodiment Construction
[0056] Exemplary embodiments of the present disclosure will be described in more detail below with reference to the accompanying drawings. Although exemplary embodiments of the present disclosure are shown in the drawings, it should be understood that the present disclosure may be embodied in various forms and should not be limited to the embodiments set forth herein. Rather, these embodiments are provided for more thorough understanding of the present disclosure and to fully convey the scope of the present disclosure to those skilled in the art.
[0057] refer to figure 1 As shown, the graph convolutional neural network-based reinforcement learning knowledge map reasoning method provided by the embodiment of the present invention includes the following steps:
[0058] S10. Based on the deep time series model and the graph convolutional neural network model, represent the historical path and the multi-hop neighborhood information of the entities on it, as the agent's percepti...
PUM
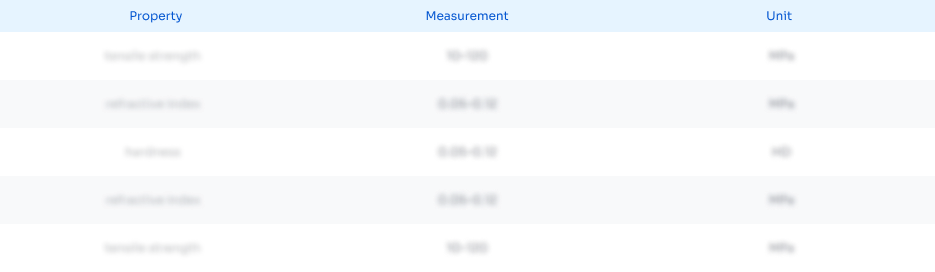
Abstract
Description
Claims
Application Information
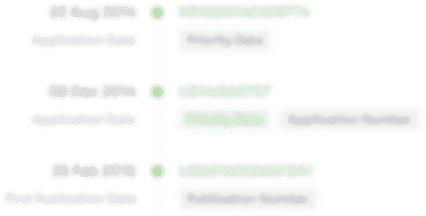
- R&D Engineer
- R&D Manager
- IP Professional
- Industry Leading Data Capabilities
- Powerful AI technology
- Patent DNA Extraction
Browse by: Latest US Patents, China's latest patents, Technical Efficacy Thesaurus, Application Domain, Technology Topic.
© 2024 PatSnap. All rights reserved.Legal|Privacy policy|Modern Slavery Act Transparency Statement|Sitemap