Group behavior recognition method based on video clip attention mechanism and interactive relation activity diagram modeling
A technology of video clips and recognition methods, which is applied in the field of group behavior recognition based on video clip attention mechanism and interactive relationship activity graph modeling, which can solve the limitation of model training data set expansion and the inability to extract underlying information dependencies and data Set doping and other issues
- Summary
- Abstract
- Description
- Claims
- Application Information
AI Technical Summary
Problems solved by technology
Method used
Image
Examples
Embodiment Construction
[0083] In order to understand the above-mentioned purpose, features and advantages of the present invention more clearly, the present invention will be further described below in conjunction with the accompanying drawings and embodiments. Many specific details are set forth in the following description to facilitate a full understanding of the present invention. However, the present invention can also be implemented in other ways than those described here. Therefore, the present invention is not limited to the specific embodiments disclosed below.
[0084] The invention discloses a group behavior recognition method based on video segment attention mechanism and interaction relationship activity graph modeling, which includes the following steps:
[0085] Step 1: Extract key video segments based on segment attention mechanism;
[0086] The second step: extract the spatio-temporal features of key video clips based on P3DResNet;
[0087] Step 3: Based on the convolution relation...
PUM
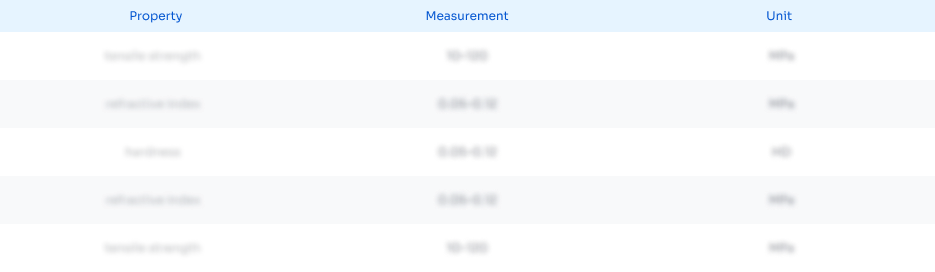
Abstract
Description
Claims
Application Information
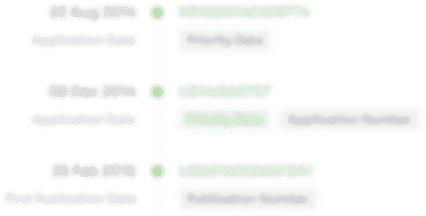
- R&D Engineer
- R&D Manager
- IP Professional
- Industry Leading Data Capabilities
- Powerful AI technology
- Patent DNA Extraction
Browse by: Latest US Patents, China's latest patents, Technical Efficacy Thesaurus, Application Domain, Technology Topic.
© 2024 PatSnap. All rights reserved.Legal|Privacy policy|Modern Slavery Act Transparency Statement|Sitemap