A compressed domain video action recognition method and system based on new spatio-temporal feature flow
An action recognition and new space-time technology, applied in the field of deep learning and pattern recognition, can solve problems such as high computational overhead, inability to handle scale changes, and low recognition accuracy, so as to avoid complete decoding and reconstruction, facilitate real-time application, and improve processing efficiency effect
- Summary
- Abstract
- Description
- Claims
- Application Information
AI Technical Summary
Problems solved by technology
Method used
Image
Examples
Embodiment Construction
[0057] In order to make the object, technical solution and advantages of the present invention clearer, the present invention will be further described in detail below in conjunction with the accompanying drawings and embodiments. It should be understood that the specific embodiments described here are only used to explain the present invention, not to limit the present invention. In addition, the technical features involved in the various embodiments of the present invention described below can be combined with each other as long as they do not constitute a conflict with each other.
[0058] The present invention proposes a compression-domain video action recognition method based on a new spatio-temporal feature stream, which combines computer vision and compressed-domain video, proposes applying the traditional compression-domain preprocessing method to deep learning, and creates a new spatio-temporal feature stream in the compressed domain , using a convolutional neural net...
PUM
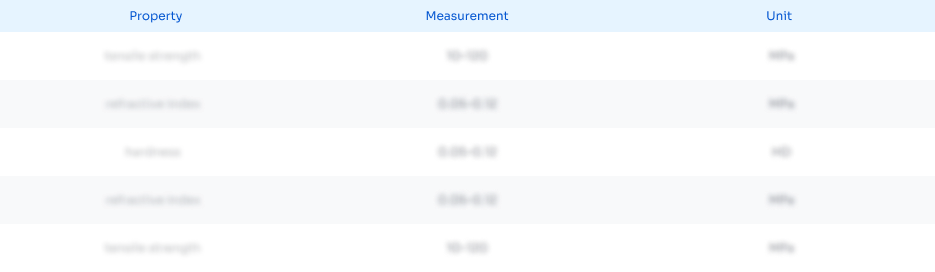
Abstract
Description
Claims
Application Information
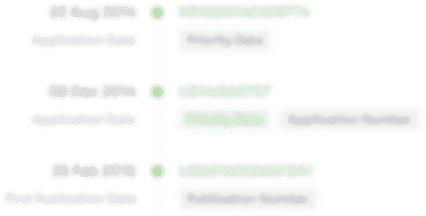
- R&D
- Intellectual Property
- Life Sciences
- Materials
- Tech Scout
- Unparalleled Data Quality
- Higher Quality Content
- 60% Fewer Hallucinations
Browse by: Latest US Patents, China's latest patents, Technical Efficacy Thesaurus, Application Domain, Technology Topic, Popular Technical Reports.
© 2025 PatSnap. All rights reserved.Legal|Privacy policy|Modern Slavery Act Transparency Statement|Sitemap|About US| Contact US: help@patsnap.com