Electric locomotive idling identification method based on empirical wavelet Hilbert transform
An empirical wavelet and electric locomotive technology, applied in character and pattern recognition, computer components, electrical digital data processing, etc., can solve problems such as ineffective use of signal frequency domain information, recognition accuracy, rapidity, or anti-interference defects , to achieve the effect of excellent recognition speed, high accuracy and strong adaptability
- Summary
- Abstract
- Description
- Claims
- Application Information
AI Technical Summary
Problems solved by technology
Method used
Image
Examples
Embodiment Construction
[0026] The present invention proposes a idling recognition method based on empirical wavelet Hilbert transform, whose input is the locomotive wheel set speed v(t) and traction torque command T b , the output is idle. The method flow chart of the present invention is as figure 1 shown, including:
[0027] Empirical wavelet transform module: Adaptive time-frequency decomposition of locomotive wheel set velocity v(t) to obtain i empirical modes v with different feature scales k (t), (k=1,2,...,i).
[0028] Optimal feature signal extraction module: by calculating the variance contribution rate to the empirical mode v of different feature scales k (t) to select, and then effectively extract the optimal feature signal v containing idling features opt (t).
[0029] Hilbert transform and its spectral analysis module: for the optimal characteristic signal v opt (t) Carry out Hilbert transform and spectral analysis to obtain the optimal characteristic signal v opt (t) Hilbert ene...
PUM
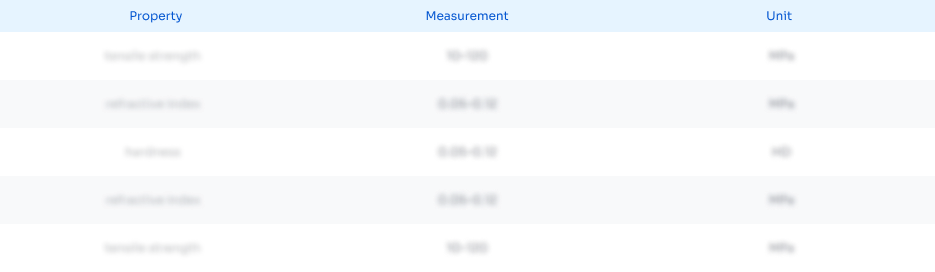
Abstract
Description
Claims
Application Information
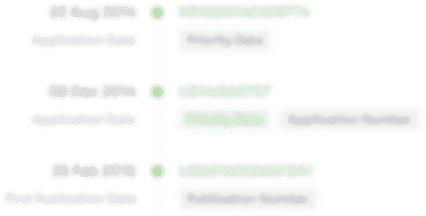
- R&D
- Intellectual Property
- Life Sciences
- Materials
- Tech Scout
- Unparalleled Data Quality
- Higher Quality Content
- 60% Fewer Hallucinations
Browse by: Latest US Patents, China's latest patents, Technical Efficacy Thesaurus, Application Domain, Technology Topic, Popular Technical Reports.
© 2025 PatSnap. All rights reserved.Legal|Privacy policy|Modern Slavery Act Transparency Statement|Sitemap|About US| Contact US: help@patsnap.com