MB-SSD method and MB-SSD feature extraction network suitable for target detection
A feature extraction and target detection technology, applied in the field of computer vision, can solve problems such as poor detection of small targets, false detection, missed detection of small targets, etc., and achieve the effect of improving detection accuracy, increasing the number of features, and speeding up learning
- Summary
- Abstract
- Description
- Claims
- Application Information
AI Technical Summary
Problems solved by technology
Method used
Image
Examples
specific Embodiment 1
[0054] combine figure 1 , the present invention mentions a kind of MB-SSD method suitable for target detection, described MB-SSD method comprises the following steps:
[0055] S1, extract multiple small target images, and use the generative confrontation network to enhance the extracted multiple small target images.
[0056] S2, constructing the MB-SSD feature extraction network, the MB-SSD feature extraction network includes a main branch feature extraction network, a branch feature extraction network and a positioning network, inputting the enhanced data into the MB-SSD feature extraction network, and obtaining the main branch features respectively Extract the classification and positioning results of the network and branch feature extraction network; then adjust the output features of the classification network according to the IoU coincidence degree of different candidate frames in the same area on the positioning network; wherein, the structure of the branch feature extra...
specific Embodiment 2
[0085] The ILSVR data set is used to pre-train the SSD main branch and branch feature extraction network, and the parameters with the best classification effect are selected as the network initialization parameters. The network is then trained using the PASCAL VOC training set.
[0086] Dataset: Use the ILSVR dataset to pre-train the SSD main branch and branch feature extraction network, and select the parameter with the best classification effect as the network initialization parameter. The network is trained using the PASCAL VOC2012 training set. Use the PASCAL VOC test set to test the detection effect.
[0087] Experimental parameters: the batch is set to 32, the momentum is set to 0.9, the learning rate adopts the exponential decay method, the initial learning rate is set to 0.01, and the decay coefficient is set to 0.9.
[0088] Experimental environment: graphics card: Nvidia GeForce RTX 2080 Ti, processor: Intel Core i7-9700K, motherboard: MSI MAG Z390 TOMAHAWK.
[00...
PUM
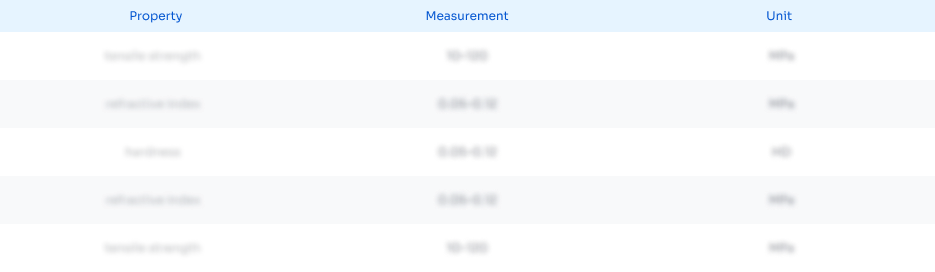
Abstract
Description
Claims
Application Information
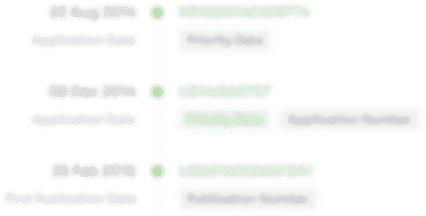
- R&D Engineer
- R&D Manager
- IP Professional
- Industry Leading Data Capabilities
- Powerful AI technology
- Patent DNA Extraction
Browse by: Latest US Patents, China's latest patents, Technical Efficacy Thesaurus, Application Domain, Technology Topic, Popular Technical Reports.
© 2024 PatSnap. All rights reserved.Legal|Privacy policy|Modern Slavery Act Transparency Statement|Sitemap|About US| Contact US: help@patsnap.com