Binocular deep learning method based on adaptive single-peak stereo matching cost filtering
A stereo matching and deep learning technology, applied in neural learning methods, image data processing, biological neural network models, etc., can solve the problem that image feature extraction and cost calculation functions cannot be effectively learned, lack, and low accuracy of binocular matching results. And other issues
- Summary
- Abstract
- Description
- Claims
- Application Information
AI Technical Summary
Problems solved by technology
Method used
Image
Examples
Embodiment Construction
[0040] The technical characteristics of the present invention will be further described below in conjunction with the accompanying drawings and specific embodiments.
[0041] As shown in the figure, the present invention is a binocular deep learning method based on adaptive unimodal stereo matching cost filtering. Ideally, the matching cost distribution of each pixel is a unimodal distribution centered on the true parallax. In order to explicitly constrain the network to learn this cost distribution to learn more robust image features and cost calculation functions, we propose to generate a unimodal cost distribution centered on the true disparity for each pixel based on the true disparity map, and use it to predict the network The matching cost body (Cost Volume) directly applies supervision. In order to reveal the matching uncertainty of each pixel, we design a confidence estimation network to estimate the confidence of each pixel and use it to adjust its corresponding true ...
PUM
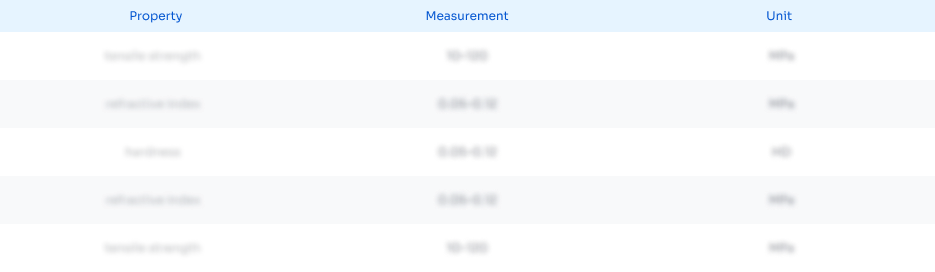
Abstract
Description
Claims
Application Information
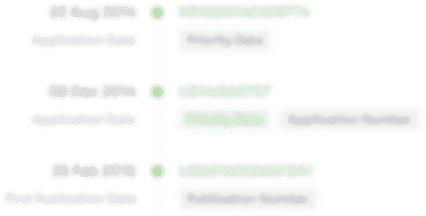
- R&D Engineer
- R&D Manager
- IP Professional
- Industry Leading Data Capabilities
- Powerful AI technology
- Patent DNA Extraction
Browse by: Latest US Patents, China's latest patents, Technical Efficacy Thesaurus, Application Domain, Technology Topic.
© 2024 PatSnap. All rights reserved.Legal|Privacy policy|Modern Slavery Act Transparency Statement|Sitemap