Multi-working-condition process monitoring method with continuous learning capability and improved PCA
A technology of process monitoring and learning ability, which is applied in complex mathematical operations, instruments, adaptive control, etc., and can solve problems such as poor real-time performance and forgetting
- Summary
- Abstract
- Description
- Claims
- Application Information
AI Technical Summary
Problems solved by technology
Method used
Image
Examples
Embodiment Construction
[0054] The specific embodiment of the present invention will be further described below in conjunction with accompanying drawing and specific embodiment:
[0055] combine figure 1 As shown, the multi-condition process monitoring method with continuous learning ability to improve PCA (principal component analysis) includes the following steps:
[0056] Step 1: Offline training, sequentially collect the data of normal operating conditions to form a training data set, use PCA to train the initial working conditions, and then use the PCA-EWC algorithm to sequentially train the subsequent working conditions, calculate the projection matrix, and build monitoring statistics Indicators and calculate thresholds, including the following steps:
[0057] a) Collect normal operating conditions Under the training data, denoted as X 1 , the sample size is N 1 , calculate the sample mean and standard deviation, and standardize the data, with a mean of 0 and a standard deviation of 1.
...
PUM
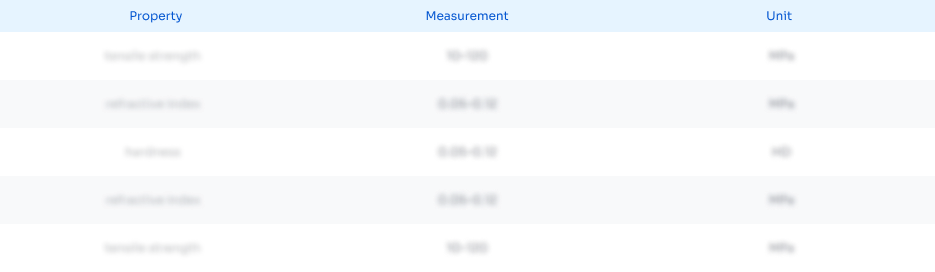
Abstract
Description
Claims
Application Information
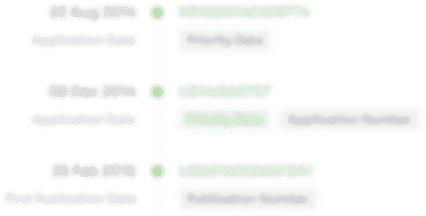
- Generate Ideas
- Intellectual Property
- Life Sciences
- Materials
- Tech Scout
- Unparalleled Data Quality
- Higher Quality Content
- 60% Fewer Hallucinations
Browse by: Latest US Patents, China's latest patents, Technical Efficacy Thesaurus, Application Domain, Technology Topic, Popular Technical Reports.
© 2025 PatSnap. All rights reserved.Legal|Privacy policy|Modern Slavery Act Transparency Statement|Sitemap|About US| Contact US: help@patsnap.com