Sorting learning method based on dual-cooperation generative adversarial network
A ranking learning and generative technology, applied in neural learning methods, biological neural network models, metadata text retrieval, etc., can solve problems such as weakening the degree of overfitting
- Summary
- Abstract
- Description
- Claims
- Application Information
AI Technical Summary
Problems solved by technology
Method used
Image
Examples
Embodiment
[0114] We conducted a benchmark test in LETOR4.0. LETOR4.0 contains 8 data sets from two query sets and four ranking settings from Gov2 webpage collection. The packaging uses a 5-fold cross-validation strategy and contains 5-fold partitions. In each fold, there are three learning subsets: training set, validation set and test set. In this example, we use MQ2007-semi and MQ2008-semi as experimental data sets.
[0115] index
[0116] Use a variety of indicators to evaluate the ranking method of information retrieval: accuracy indicators (P@3, P@5, P@10, MAP, MRR), normalized discounted cumulative gain (NDCG) These indicators are evaluated at the learning level. The accuracy metric (P@k) only considers the prediction accuracy of the first k positions, while MRR considers the position of the first positive term.
[0117] In order to summarize them, MAP was proposed to observe all positive items in a comprehensive manner. The NDCG metric considers not only the correlation, but also t...
PUM
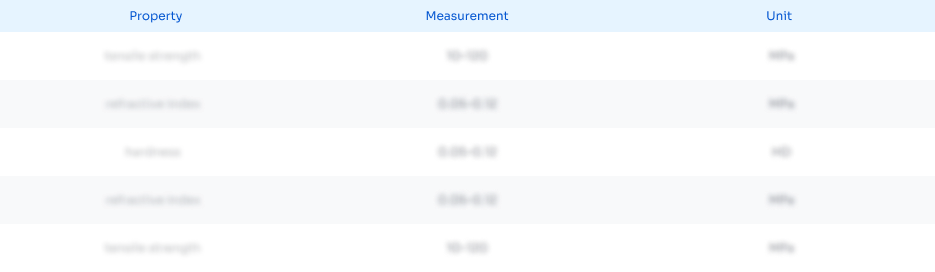
Abstract
Description
Claims
Application Information
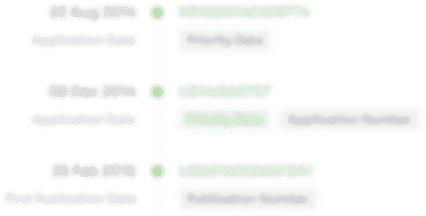
- R&D Engineer
- R&D Manager
- IP Professional
- Industry Leading Data Capabilities
- Powerful AI technology
- Patent DNA Extraction
Browse by: Latest US Patents, China's latest patents, Technical Efficacy Thesaurus, Application Domain, Technology Topic, Popular Technical Reports.
© 2024 PatSnap. All rights reserved.Legal|Privacy policy|Modern Slavery Act Transparency Statement|Sitemap|About US| Contact US: help@patsnap.com