Implementing method of sliding directional drilling simulator
A realization method, a technology of directional drilling, which is applied in directional drilling, earthwork drilling, wellbore/well components, etc., and can solve problems such as high cost, large error, and long installation period
- Summary
- Abstract
- Description
- Claims
- Application Information
AI Technical Summary
Problems solved by technology
Method used
Image
Examples
Embodiment 1
[0035] A kind of sliding directional drilling simulator realization method based on GAN that the present invention relates to, such as figure 1 shown, including the following steps:
[0036] (1) Obtain the sliding orientation training data set, and generate random noise at the same time;
[0037] (2) Carry out data processing and data sorting, and generate a model to generate data samples using random noise;
[0038] (3) Use the discriminant model to judge the data source, generate an confrontation network (GAN), and form a sliding directional data model;
[0039] (4) Automatically generate multi-category sliding orientation data, obtain effective amplified data, and form an amplified sliding data set.
[0040] In step (1), the acquired sliding orientation training data set contains at least the data of three systems including torsion pendulum, MWD and mud logging system.
[0041] In step (1), the random noise is equivalent to a random variable, as the input data of the gen...
Embodiment 2
[0053] This embodiment combines the attached figure 2 The calculation flow of the GAN of the present invention will be described.
[0054] Such as figure 2 Shown:
[0055] In the first step, any differentiable function can be used to represent the generative model and discriminant model of GAN. The differentiable functions D and G are used to represent the discriminant model and the generative model respectively. The input of D is the real data x and G(z), The input of G is a random variable z. Among them, G(z) is a data sample generated by G that obeys the real data distribution as much as possible.
[0056] In the second step, if the input of the discriminant model comes from real data, it is marked as 1, and if the input sample is G(z), it is marked as 0.
[0057] In the third step, the goal of D is to realize the two-category discrimination of the data source, and judge whether the data is true (the distribution of real data x) or false (the false data G(z) of the ge...
Embodiment 3
[0060] The sliding orientation training data set is regarded as composed of multiple data points. When processing the sliding orientation training data set, the n×m dimensional "data block" divided by the processed effective data set can be compared to the pixels of the picture Point matrix, each data in the data block is a data step. Then, through the training of the network model combining the convolutional neural network and the generative confrontation network, the purpose of expanding the sequence data sample set can be achieved. Its overall model is as image 3 shown.
[0061] The generative model network structure of the generative confrontation network is as follows: Figure 4 shown. A high-dimensional random noise vector is input into the generation model G, and spatial upsampling is performed through three layers of micro-step convolution layers. The number of channels of the input data is halved, the size of the "data block" is doubled, and finally a 64×64×3 of ...
PUM
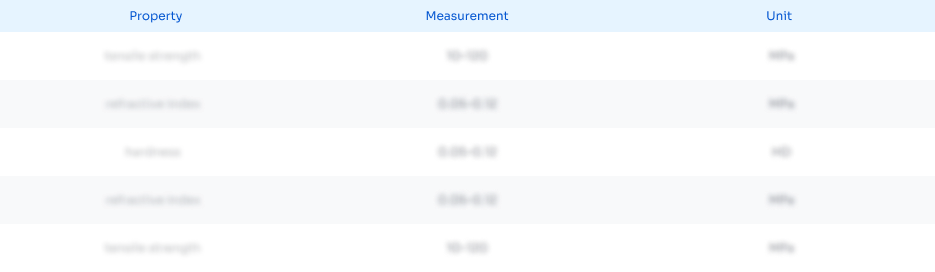
Abstract
Description
Claims
Application Information
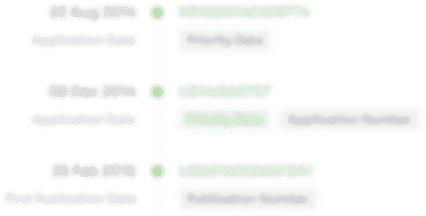
- R&D Engineer
- R&D Manager
- IP Professional
- Industry Leading Data Capabilities
- Powerful AI technology
- Patent DNA Extraction
Browse by: Latest US Patents, China's latest patents, Technical Efficacy Thesaurus, Application Domain, Technology Topic, Popular Technical Reports.
© 2024 PatSnap. All rights reserved.Legal|Privacy policy|Modern Slavery Act Transparency Statement|Sitemap|About US| Contact US: help@patsnap.com