Efficient lifelong relationship extraction method and system based on dynamic regularization
A technology of relation extraction and relation, applied in neural learning methods, instruments, biological neural network models, etc., can solve the problems of expensive process, inability to adapt models, and time-consuming
- Summary
- Abstract
- Description
- Claims
- Application Information
AI Technical Summary
Problems solved by technology
Method used
Image
Examples
Embodiment 1
[0058] refer to figure 1 , is a schematic structural diagram of an efficient lifelong relationship extraction system based on dynamic regularization in the present invention, specifically, an efficient lifelong relationship extraction system based on dynamic regularization, including:
[0059] The training module 1 is used to receive multiple data sets, and sequentially train the training samples in the data sets through the neural model, each data set corresponds to a task; the training samples include entity-to-sentence and candidate relationship sets, and real relationship labels;
[0060] Regularization module 2, establishes memory blocks to store memory data for the trained data set, and accesses the memory data of all memory blocks during training of the new data set, defines multiple loss functions at the same time, and calculates each loss function in different tasks The regularization factor between;
[0061] In this embodiment, the regularization module 2 includes a...
Embodiment 2
[0080] refer to figure 2 , is a process flow of an efficient lifelong relationship extraction method based on dynamic regularization in the present invention, specifically, an efficient lifelong relationship extraction method based on dynamic regularization, comprising the following steps:
[0081] S400: Receive multiple data sets, use the neural model to sequentially train the training samples in the data sets, each data set corresponds to a task; then execute step S500;
[0082] In this example, from a series of data sets {D 1 ,D 2 ,...,D N}, where each dataset corresponds to a task. The data for task k consists of observations and marker pairs Ideally, if all task data were available at the same time, the model could use them simultaneously for joint training, however, with standard lifelong learning setups, datasets arrive sequentially, so only one of them can be accessed at a time.
[0083] The kth task in this embodiment (ie the kth data set D k ) training sample...
Embodiment 3
[0132] In this example, the effectiveness of the system in Example 1 and the method in Example 2 is verified through experiments. Specifically, the Lifelong FewRel dataset and the Lifelong SimpleQuestions dataset are used for evaluation. The Lifelong FewRel dataset consists of 10 tasks , these tasks are obtained by dividing the FewRel dataset into 10 disjoint clusters, FewRel has a total of 80 relations, so each cluster contains 8 relations, and each sample in the cluster includes a target relation Sentences and a candidate set selected by random sampling; LifelongSimpleQuestions is constructed similarly, consisting of 20 tasks generated from the SimpleQuestions dataset.
[0133] Preferably, ACC is used in this embodiment avg and ACC whole Two metrics are used to evaluate our model. ACC avg Estimates the average test accuracy on observed tasks; ACC whole Evaluate the overall performance of the model on observed and unobserved tasks.
[0134]At the same time, the following...
PUM
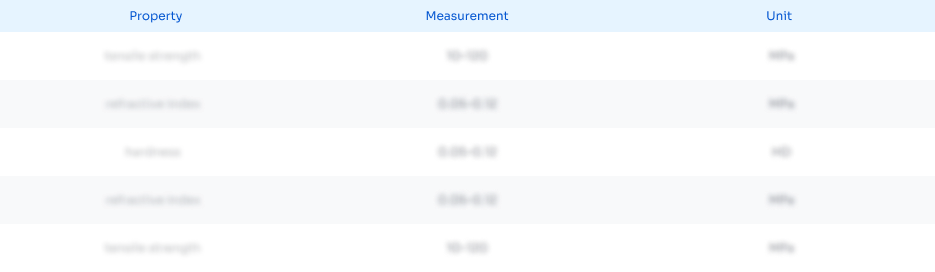
Abstract
Description
Claims
Application Information
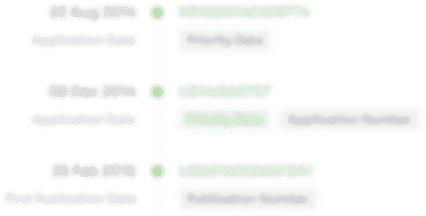
- Generate Ideas
- Intellectual Property
- Life Sciences
- Materials
- Tech Scout
- Unparalleled Data Quality
- Higher Quality Content
- 60% Fewer Hallucinations
Browse by: Latest US Patents, China's latest patents, Technical Efficacy Thesaurus, Application Domain, Technology Topic, Popular Technical Reports.
© 2025 PatSnap. All rights reserved.Legal|Privacy policy|Modern Slavery Act Transparency Statement|Sitemap|About US| Contact US: help@patsnap.com