Automatic driving vehicle microscopic decision-making method based on reinforcement learning
A technology of automatic driving and reinforcement learning, applied in the direction of neural learning methods, biological neural network models, control devices, etc., can solve the problem of difficult urban roads showing better decision-making performance, not well adapted to dynamic changes in the environment, state space and Large behavioral space and other issues, to achieve the effects of strong universality and portability, easy deployment, and strong feasibility
- Summary
- Abstract
- Description
- Claims
- Application Information
AI Technical Summary
Problems solved by technology
Method used
Image
Examples
Embodiment Construction
[0064] The present invention will be further described in detail below in conjunction with the accompanying drawings and specific embodiments.
[0065] Such as Figure 1 to Figure 4 As shown, the self-driving vehicle micro-decision-making method includes the following steps:
[0066] Step 1, reinforcement learning modeling, modeling and representation of automatic driving decision-making scheme:
[0067] In step 1.1, the driving process of the vehicle is defined as a Markov decision process. The autonomous vehicle is regarded as an agent, and the driving environment of the vehicle is regarded as a reinforcement learning environment. The agent vehicle makes driving decisions and Driving behavior, adjust the driving decision based on the driving results, divide the driving time into multiple time slots, each agent vehicle makes a driving decision at the beginning of the time slot, and determine the driving behavior of each agent vehicle in the time slot;
[0068] Step 1.2, use...
PUM
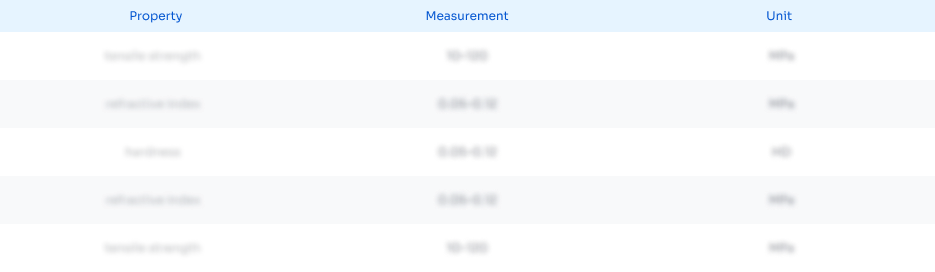
Abstract
Description
Claims
Application Information
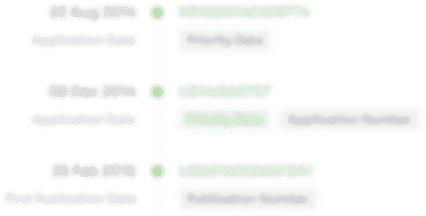
- R&D
- Intellectual Property
- Life Sciences
- Materials
- Tech Scout
- Unparalleled Data Quality
- Higher Quality Content
- 60% Fewer Hallucinations
Browse by: Latest US Patents, China's latest patents, Technical Efficacy Thesaurus, Application Domain, Technology Topic, Popular Technical Reports.
© 2025 PatSnap. All rights reserved.Legal|Privacy policy|Modern Slavery Act Transparency Statement|Sitemap|About US| Contact US: help@patsnap.com