Myoelectric gesture recognition method based on multi-feature fusion CNN
A multi-feature fusion and gesture recognition technology, applied in the field of physiological signal recognition, can solve the problems of short model training time, loss of original signal feature information, cumbersome and complicated work, etc.
- Summary
- Abstract
- Description
- Claims
- Application Information
AI Technical Summary
Problems solved by technology
Method used
Image
Examples
Embodiment Construction
[0022] The technical solution of the present invention will be further described in detail below in conjunction with the accompanying drawings.
[0023] Aiming at the current problems, the present invention proposes a gesture recognition method based on parallel feature fusion convolutional neural network architecture from the perspective of reducing feature set dependence and optimizing training process features. This method proposes a parallel-based feature fusion convolutional neural network architecture. In order to simplify the training process and reduce the dependence of the training set on the design of a specific feature set, the method is designed based on the convolutional neural network architecture in the deep learning method; secondly, the parallel neural network is used in the architecture to analyze the time domain signal and the frequency domain signal. Simultaneous training helps to improve training efficiency and reduce training time; in the training model, ...
PUM
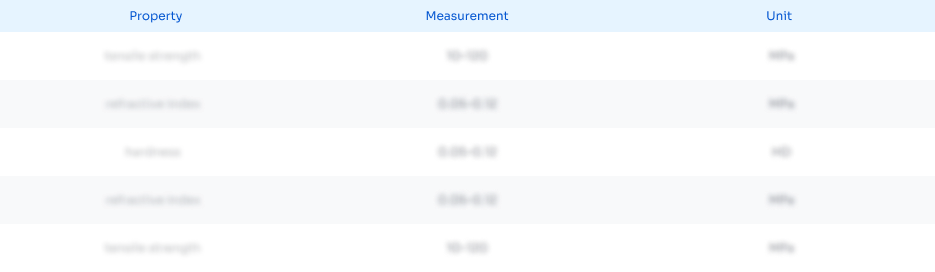
Abstract
Description
Claims
Application Information
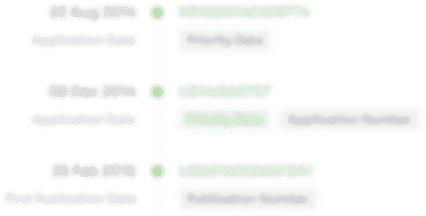
- R&D
- Intellectual Property
- Life Sciences
- Materials
- Tech Scout
- Unparalleled Data Quality
- Higher Quality Content
- 60% Fewer Hallucinations
Browse by: Latest US Patents, China's latest patents, Technical Efficacy Thesaurus, Application Domain, Technology Topic, Popular Technical Reports.
© 2025 PatSnap. All rights reserved.Legal|Privacy policy|Modern Slavery Act Transparency Statement|Sitemap|About US| Contact US: help@patsnap.com