Large-scale MIMO downlink precoding method based on deep learning
A deep learning and precoding technology, applied in the field of massive MIMO downlink precoding, can solve problems such as difficult optimal solution, ignoring instantaneous CSI, channel outdated, etc., to achieve efficient calculation and reduce computational complexity.
- Summary
- Abstract
- Description
- Claims
- Application Information
AI Technical Summary
Problems solved by technology
Method used
Image
Examples
Embodiment Construction
[0031] In order to enable those skilled in the art to better understand the solutions of the present invention, the technical solutions in the embodiments of the present invention will be clearly and completely described below in conjunction with the drawings in the embodiments of the present invention.
[0032]In the massive MIMO downlink precoding method based on deep learning disclosed in the embodiment of the present invention, the base station is equipped with a large-scale one-dimensional or two-dimensional antenna array, and uses the instantaneous and statistical channel state information of each user terminal to reach Rate or its approximation utility function maximization criterion, calculate the precoding vector corresponding to each user terminal through a general framework or a low complexity framework, and then use the obtained vector for downlink precoding transmission; during the mobile process of the user terminal In , as the instantaneous and statistical channe...
PUM
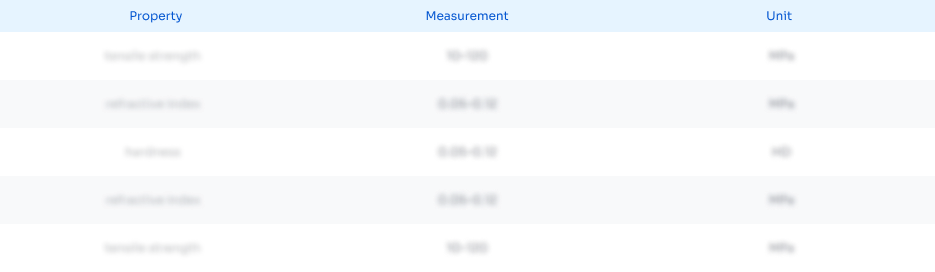
Abstract
Description
Claims
Application Information
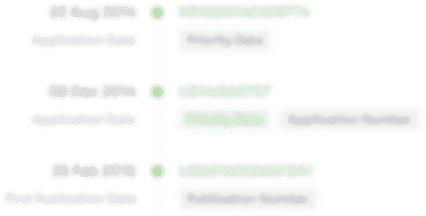
- R&D Engineer
- R&D Manager
- IP Professional
- Industry Leading Data Capabilities
- Powerful AI technology
- Patent DNA Extraction
Browse by: Latest US Patents, China's latest patents, Technical Efficacy Thesaurus, Application Domain, Technology Topic, Popular Technical Reports.
© 2024 PatSnap. All rights reserved.Legal|Privacy policy|Modern Slavery Act Transparency Statement|Sitemap|About US| Contact US: help@patsnap.com