Intelligent fault diagnosis method based on deep adversarial domain self-adaption
A domain self-adaptive and fault diagnosis technology, applied in neural learning methods, instruments, biological neural network models, etc., can solve problems such as performance deterioration of intelligent diagnosis methods
- Summary
- Abstract
- Description
- Claims
- Application Information
AI Technical Summary
Problems solved by technology
Method used
Image
Examples
Embodiment Construction
[0066] In order to more clearly illustrate the embodiments of the present invention or the technical solutions in the prior art, the accompanying drawings used in the embodiments will be briefly introduced below. Obviously, the accompanying drawings in the following description are only some implementations of the present invention For example, those skilled in the art can also obtain other drawings based on these drawings without creative work.
[0067] The present invention will be further described in detail below in conjunction with specific examples, which are explanations rather than limitations of the present invention.
[0068] refer to figure 1 As shown, the present invention provides an intelligent fault diagnosis method based on deep confrontation domain self-adaptation: use sensors to collect vibration signals of various states of rotating machinery under different working conditions, and use moving time windows to perform signal processing on data sets under diffe...
PUM
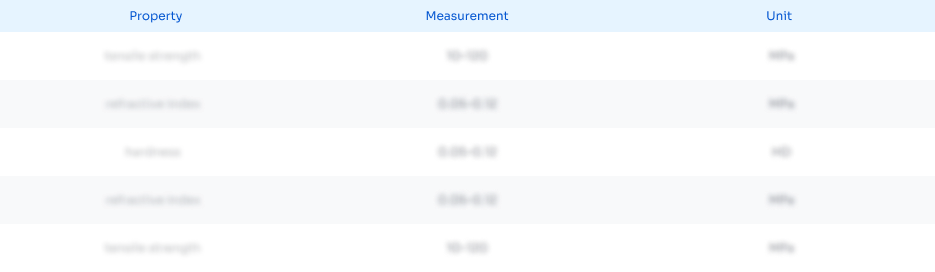
Abstract
Description
Claims
Application Information
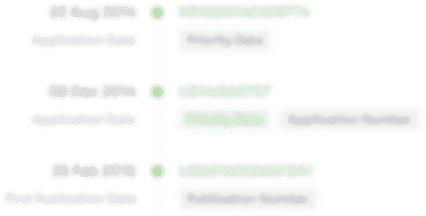
- R&D Engineer
- R&D Manager
- IP Professional
- Industry Leading Data Capabilities
- Powerful AI technology
- Patent DNA Extraction
Browse by: Latest US Patents, China's latest patents, Technical Efficacy Thesaurus, Application Domain, Technology Topic, Popular Technical Reports.
© 2024 PatSnap. All rights reserved.Legal|Privacy policy|Modern Slavery Act Transparency Statement|Sitemap|About US| Contact US: help@patsnap.com