Industrial control system intrusion detection method based on small sample learning
A technology for industrial control systems and intrusion detection, applied in neural learning methods, transmission systems, machine learning, etc., can solve problems such as samples that cannot distinguish new types of attacks, complex structures, and difficulty in detecting attack types
- Summary
- Abstract
- Description
- Claims
- Application Information
AI Technical Summary
Problems solved by technology
Method used
Image
Examples
Embodiment 1
[0084] The present invention designs an industrial control system intrusion detection method based on small sample learning, comprising the following steps:
[0085] 1) Data set division. The three different types of samples of the original data set extracted from the data flow of the industrial control system are divided into a detection model training set and a basic model training set. The three different types of samples are normal samples and common attack samples. samples and new attack samples;
[0086] 2) Continuous data processing, using Principal Component Analysis (PCA) to analyze the basic model training set and detection model training set of data samples Data Matrix Constructed by Dimensional Continuous Features Carry out dimensionality reduction;
[0087] 3) Discrete data processing, use one-hot encoding (One-hot Encoding) method for basic model training set, detection model training set of data samples A data matrix composed of discrete features proce...
Embodiment 2
[0093] This embodiment is further optimized on the basis of the above embodiments, and the same parts as the aforementioned technical solutions will not be repeated here. Further, in order to better realize the present invention, the following setting methods are specially adopted: the steps 1) specifically is: will contain a large number of normal samples of normal types, common attack samples of at least 3 common attack types and A new attack sample of a new attack type ( ) The original data sets of three different types of samples are divided in the following way to obtain the detection model training set and the basic model training set:
[0094] 1.1) Extract from normal samples and common attack samples of a common attack type to be detected samples, with A new attack sample constitutes the detection model training set;
[0095] 1.2) The remaining normal samples and common attack samples of common attack types are used to form the basic model training set.
Embodiment 3
[0097] This embodiment is further optimized on the basis of any of the above-mentioned embodiments, and the same parts as the aforementioned technical solutions will not be repeated here. Further, in order to better realize the present invention, the following setting methods are adopted in particular: the step 2 ) The specific steps are:
[0098] 2.1) Use the z-score standardization method for the basic model training set and the detection model training set of data samples A data matrix composed of continuous features For preprocessing, the data matrix B in the column data data According to formula (1), it is transformed into :
[0099] (1),
[0100] in, , respectively represent the The mean and standard deviation of the column data;
[0101] 2.2) After preprocessing, calculate the data matrix according to formula (2) The optimal eigenmatrix of :
[0102] (2),
[0103] in, is the data matrix The characteristic matrix of the dimension is ...
PUM
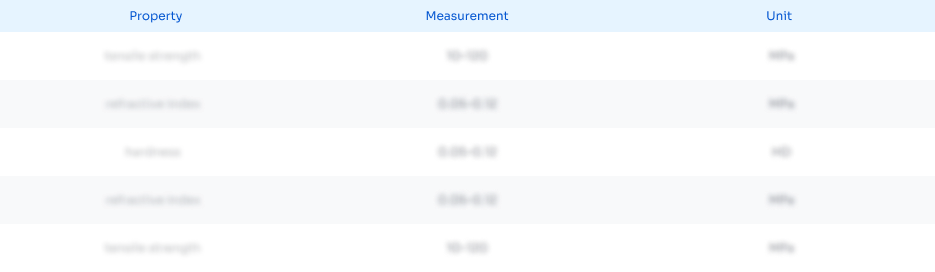
Abstract
Description
Claims
Application Information
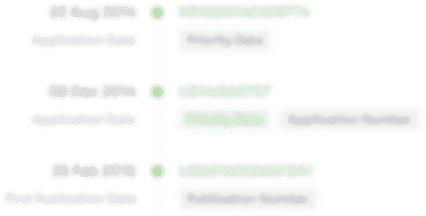
- R&D Engineer
- R&D Manager
- IP Professional
- Industry Leading Data Capabilities
- Powerful AI technology
- Patent DNA Extraction
Browse by: Latest US Patents, China's latest patents, Technical Efficacy Thesaurus, Application Domain, Technology Topic, Popular Technical Reports.
© 2024 PatSnap. All rights reserved.Legal|Privacy policy|Modern Slavery Act Transparency Statement|Sitemap|About US| Contact US: help@patsnap.com