Freight supervision abnormal information collection method and device, server and storage medium
A technology of abnormal information and acquisition methods, which is applied in the direction of instruments, relational databases, data processing applications, etc., can solve problems such as ignoring relationships, affecting the mining of abnormal behavior patterns of enterprises, and appearing meaningless, achieving high efficiency and intuitive and accurate results. sex high effect
- Summary
- Abstract
- Description
- Claims
- Application Information
AI Technical Summary
Problems solved by technology
Method used
Image
Examples
Embodiment 1
[0032] figure 1 It is a flow chart of the method for obtaining freight supervision abnormal information in Embodiment 1 of the present invention. The method can be executed by a terminal or a server. In this embodiment, a terminal is taken as an example. The method specifically includes:
[0033] S110, extracting triplets based on freight supervision data to generate a knowledge network graph.
[0034] Freight supervision data includes text data and table data recorded in the supervision of the cargo transportation process. Triplets refer to data sets in the form of "entity A-[relation R]->entity B" and "entity A-attribute category-attribute value", which are suitable for mining relational networks, where "entity A-[relation R] ]->Entity B" indicates the relationship between entities, and "Entity A-Attribute Category-Attribute Value" indicates the attribute of a single entity. The knowledge network graph is a graph structure that represents the entity attributes and the rela...
Embodiment 2
[0047] This embodiment further supplements some content on the basis of Embodiment 1 to further explain some steps, specifically including:
[0048] Such as figure 2 As shown, step S110 specifically includes steps S111-112:
[0049] S111. Extract entities based on freight supervision data, and create nodes according to the entities.
[0050] In this embodiment, the data stored in the knowledge network map is the NEO4J graph database. For the initial full amount of data, NEO4J-impot will be used to import the node attribute and association relationship triplet data using csv. Each column of the node attribute class csv contains all the attribute types of the entity that need to be imported into NEO4J. The first column has a mark like ":ID(Entity)", ID represents the primary key of the column, and the value of the Entity in the brackets is the The entity name of the class entity. The csv file of the association relationship type contains at least two columns, which need to c...
Embodiment 3
[0077] Figure 6 The device 300 for obtaining freight supervision abnormality information provided by Embodiment 3 of the present invention specifically includes the following modules:
[0078]A data extraction module 310, configured to extract triples based on freight supervision data to generate a knowledge network map;
[0079] The subgraph query module 320 is used to obtain corresponding multiple subgraph query results through multiple preset subgraph query models based on the knowledge network map, and each subgraph query result includes one or more subgraphs;
[0080] A support calculation module 330, configured to determine the support of each subgraph query result;
[0081] A subgraph query result screening module 340, configured to determine that the subgraph query result whose support degree is greater than a support threshold is a target subgraph query result;
[0082] The abnormal information determination module 350 is configured to determine the confidence leve...
PUM
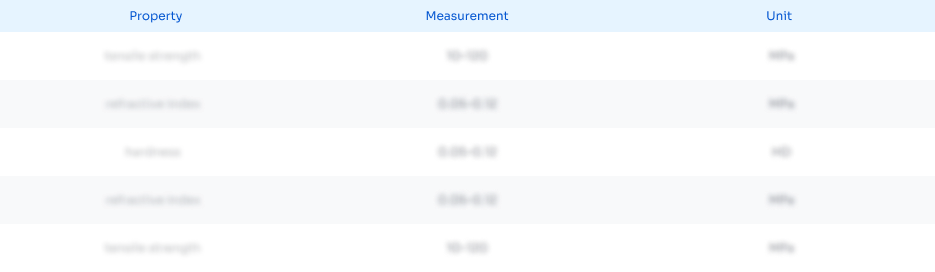
Abstract
Description
Claims
Application Information
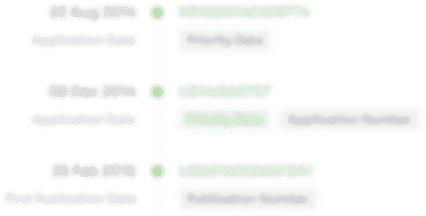
- R&D
- Intellectual Property
- Life Sciences
- Materials
- Tech Scout
- Unparalleled Data Quality
- Higher Quality Content
- 60% Fewer Hallucinations
Browse by: Latest US Patents, China's latest patents, Technical Efficacy Thesaurus, Application Domain, Technology Topic, Popular Technical Reports.
© 2025 PatSnap. All rights reserved.Legal|Privacy policy|Modern Slavery Act Transparency Statement|Sitemap|About US| Contact US: help@patsnap.com