Cluster resource management and task scheduling method and system based on deep reinforcement learning
A technology of reinforcement learning and task scheduling, applied in neural learning methods, resource allocation, electrical digital data processing, etc., can solve problems such as inability to take into account multiple goals at the same time, low utilization of system resources, and inability to achieve box packing effects, etc. Achieve the effects of reducing the number of resource fragments, improving system efficiency, and saving manpower
- Summary
- Abstract
- Description
- Claims
- Application Information
AI Technical Summary
Problems solved by technology
Method used
Image
Examples
Embodiment Construction
[0027] In order to make the above objects, features and advantages of the present invention more comprehensible, the present invention will be further described in detail below through specific embodiments and accompanying drawings.
[0028] Such as figure 1 As shown, the present invention mainly includes a task queue to be scheduled, a resource scheduling management agent, a task scheduling module and a simulator, and is mainly divided into two processes of training and running.
[0029] The training process is to collect task running conditions for a period of time on the computer cluster, including task resource demand vectors, task request time and other attributes. Based on the collected data, in the simulator for figure 2 The Q neural network shown is trained until the expected goal is reached, then the training ends.
[0030] After the training will be figure 2 The Q neural network parameters shown are copied to figure 1 The resource scheduling management agent in...
PUM
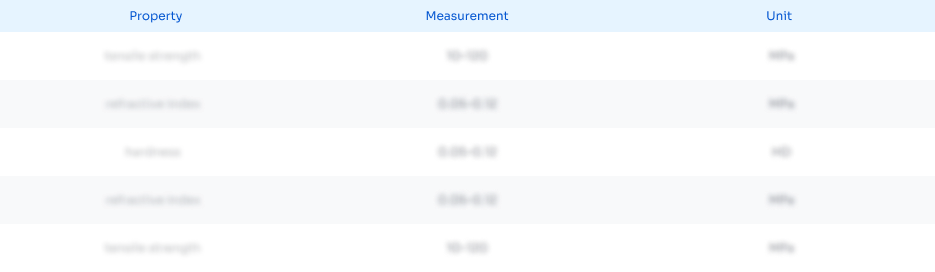
Abstract
Description
Claims
Application Information
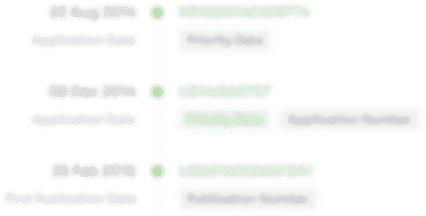
- R&D
- Intellectual Property
- Life Sciences
- Materials
- Tech Scout
- Unparalleled Data Quality
- Higher Quality Content
- 60% Fewer Hallucinations
Browse by: Latest US Patents, China's latest patents, Technical Efficacy Thesaurus, Application Domain, Technology Topic, Popular Technical Reports.
© 2025 PatSnap. All rights reserved.Legal|Privacy policy|Modern Slavery Act Transparency Statement|Sitemap|About US| Contact US: help@patsnap.com