Permanent magnet synchronous motor fault diagnosis method based on deep learning
A technology of permanent magnet synchronous motor and deep learning, applied in the direction of neural learning method, motor generator test, electric winding test, etc. Sensor synthesis, multi-feature fusion, etc.
- Summary
- Abstract
- Description
- Claims
- Application Information
AI Technical Summary
Problems solved by technology
Method used
Image
Examples
Embodiment Construction
[0023] Specific embodiments of the present invention will be described below in conjunction with the accompanying drawings, so that those skilled in the art can better understand the present invention. It should be noted that in the following description, when detailed descriptions of known functions and designs may dilute the main content of the present invention, these descriptions will be omitted here.
[0024] The Hall current sensor, piezoelectric acceleration sensor and microphone are used to collect the stator current signal, vibration signal and noise signal of the permanent magnet synchronous motor, and at least 2000 1s-long signals are collected for each fault.
[0025] Signal preprocessing uses the method of wavelet denoising to filter, and then calculates the time domain characteristics of the signal, except the peak value and mean value RMS X rms In addition, there are:
[0026] 1) Peak indicator:
[0027]
[0028] 2) Kurtosis index:
[0029]
[0030] 3...
PUM
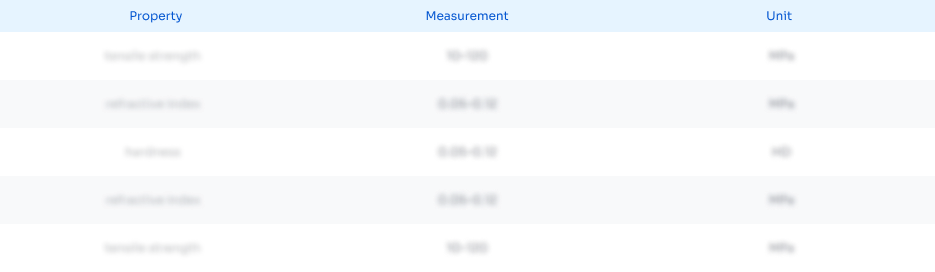
Abstract
Description
Claims
Application Information
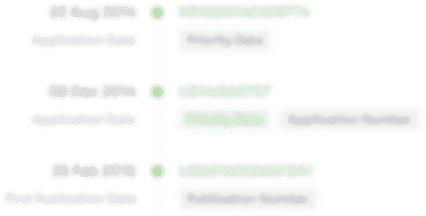
- R&D Engineer
- R&D Manager
- IP Professional
- Industry Leading Data Capabilities
- Powerful AI technology
- Patent DNA Extraction
Browse by: Latest US Patents, China's latest patents, Technical Efficacy Thesaurus, Application Domain, Technology Topic, Popular Technical Reports.
© 2024 PatSnap. All rights reserved.Legal|Privacy policy|Modern Slavery Act Transparency Statement|Sitemap|About US| Contact US: help@patsnap.com