Medical image segmentation method and device based on multi-modal subspace clustering
A medical image and clustering method technology, which is applied in image analysis, neural learning methods, image enhancement, etc., can solve the problems of good segmentation effect, high precision, and low segmentation accuracy of complex medical images
- Summary
- Abstract
- Description
- Claims
- Application Information
AI Technical Summary
Problems solved by technology
Method used
Image
Examples
Embodiment 1
[0084] Such as Figure 1 to Figure 10 As shown, the present invention is based on the medical image segmentation method of self-supervised multimodal deep subspace clustering, the segmentation method framework is as follows figure 2 As shown, the present invention builds a model based on self-supervised multimodal deep subspace clustering method, uses useful self-supervised information in subspace clustering results to guide feature learning, and refines the self-expression model. Inspired by the deep multimodal subspace clustering algorithm, on the basis of the automatic encoding network, the feature fusion is performed after the feature extraction of the data of each modality; and then the self-expression coefficient is learned through the introduction of the self-expression layer; In particular, we will introduce a dual self-supervision that integrates feature extraction for multimodal encoding modules, affinity learning for self-representation models, and data segmentatio...
Embodiment 2
[0109] Such as Figure 1 to Figure 10 As shown, the difference between this embodiment and Embodiment 1 is that this embodiment provides a medical image segmentation device, including:
[0110] Input module: used to input the acquired original medical image;
[0111] Preprocessing module: used to preprocess the original medical image input by the input unit and send it to the processing unit;
[0112] Processing module: including a first processing unit, a second processing unit and a third processing unit;
[0113] The first processing unit is configured to perform convolution and pooling on the original medical image preprocessed by the preprocessing module through a convolutional neural network, and convert it into a linear feature matrix of the original medical image;
[0114] The second processing unit is used to construct a model based on the self-supervised multimodal deep subspace clustering method, and perform model training; using the trained self-supervised multim...
Embodiment 3
[0119] Such as Figure 1 to Figure 10 As shown, the difference between this embodiment and Embodiment 1 is that this embodiment provides a medical image segmentation device, including:
[0120] memory for storing computer programs;
[0121] A processor, configured to implement the steps of the medical image segmentation method described in Embodiment 1 when executing the computer program.
PUM
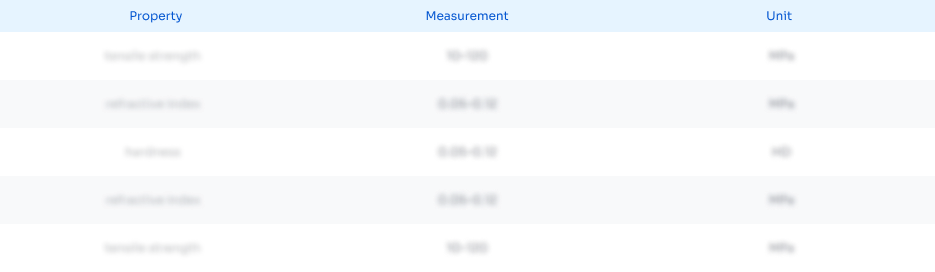
Abstract
Description
Claims
Application Information
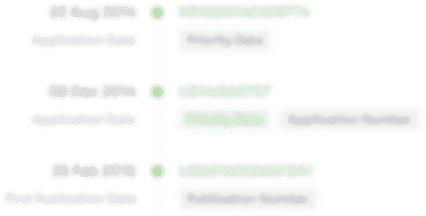
- R&D Engineer
- R&D Manager
- IP Professional
- Industry Leading Data Capabilities
- Powerful AI technology
- Patent DNA Extraction
Browse by: Latest US Patents, China's latest patents, Technical Efficacy Thesaurus, Application Domain, Technology Topic, Popular Technical Reports.
© 2024 PatSnap. All rights reserved.Legal|Privacy policy|Modern Slavery Act Transparency Statement|Sitemap|About US| Contact US: help@patsnap.com