Aerospace engine abnormity intelligent detection method based on hierarchical adversarial training
An aerospace engine and intelligent detection technology, applied in neural learning methods, computer components, complex mathematical operations, etc., can solve the difficulties of collecting abnormal data, the difficulty of engine failure mode diversity fault simulation, and the difficulty of comprehensively evaluating engine health status, etc. question
- Summary
- Abstract
- Description
- Claims
- Application Information
AI Technical Summary
Problems solved by technology
Method used
Image
Examples
Embodiment Construction
[0056]The application will be further described in detail below in conjunction with the accompanying drawings and embodiments, so that those skilled in the art can better understand the present invention. It can be understood that the specific embodiments described here are only used to explain the related invention, but not to limit the invention. For ease of description, only the parts related to the relevant invention are shown in the drawings. It should also be noted that the embodiments in the application and the features in the embodiments can be combined with each other if there is no conflict. Hereinafter, the present application will be described in detail with reference to the drawings and in conjunction with embodiments.
[0057]An aerospace engine anomaly intelligent detection method based on hierarchical confrontation training, seefigure 1 , Including the following steps:
[0058]Step 1: Use the multi-channel raw signals collected by multiple sensors as the multi-source data ...
PUM
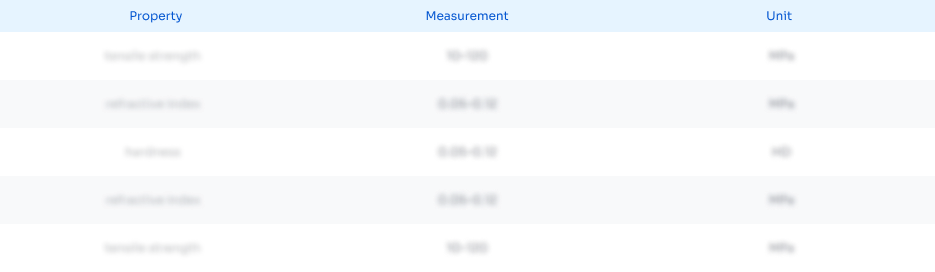
Abstract
Description
Claims
Application Information
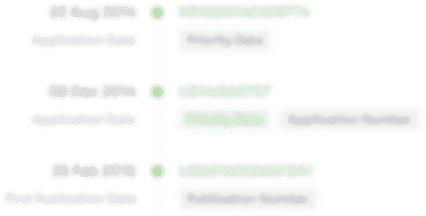
- R&D Engineer
- R&D Manager
- IP Professional
- Industry Leading Data Capabilities
- Powerful AI technology
- Patent DNA Extraction
Browse by: Latest US Patents, China's latest patents, Technical Efficacy Thesaurus, Application Domain, Technology Topic, Popular Technical Reports.
© 2024 PatSnap. All rights reserved.Legal|Privacy policy|Modern Slavery Act Transparency Statement|Sitemap|About US| Contact US: help@patsnap.com