Method and device for generating small adversarial patches
A patch and small technology, applied in the field of machine learning, can solve the problem of small feature difference and performance loss
- Summary
- Abstract
- Description
- Claims
- Application Information
AI Technical Summary
Problems solved by technology
Method used
Image
Examples
Embodiment 1
[0050] see figure 1 and figure 2 , providing a small-scale anti-patch generation method, which can be used for products or projects that generate anti-patch based on neural network gradient descent. The small-scale anti-patch generation method includes the following steps:
[0051] S1. Given an adversarial patch image, randomly initialize the adversarial patch image;
[0052] S2. Add the initialized confrontation patch image to the pasting area selected on the target object in the training data, and make it into a confrontation sample;
[0053] S3. Sending the adversarial samples into the deep learning model for extracting adversarial features, and sending benign samples without added adversarial patch images into the deep learning model for extracting benign features;
[0054] S4. Input the adversarial features and the benign features together into a feature enhancement loss function to perform loss calculation, and obtain a loss result;
[0055] S5. Add the loss result t...
Embodiment 2
[0095] see image 3 , providing a small-scale anti-patch generation device, using embodiment 1 or any possible implementation of the small-scale anti-patch generation method, including:
[0096] The anti-patch initialization module 1 is used to randomly initialize the anti-patch image for a given anti-patch image;
[0097] The confrontation sample generation module 2 is used to add the initialized confrontation patch image to the pasting area selected on the target object object in the training data, and make it into a confrontation sample;
[0098]The confrontation feature extraction module 3 is used to transport the confrontation sample into the deep learning model for confrontation feature extraction;
[0099] The benign feature extraction module 4 is used to feed the benign samples without added confrontation patch images into the deep learning model for benign feature extraction;
[0100] The loss result acquisition module 5 is used to jointly input the confrontation fe...
Embodiment 3
[0106] A computer-readable storage medium is provided, wherein the computer-readable storage medium stores program codes for Deepfake detection generated by small-scale anti-patches, and the program codes are included in the implementation of Embodiment 1 or any possible implementation thereof. Instructions for small adversarial patch generation methods.
[0107] The computer-readable storage medium may be any available medium that can be accessed by a computer, or a data storage device such as a server, a data center, etc. integrated with one or more available media. The available medium may be a magnetic medium (for example, a floppy disk, a hard disk, or a magnetic tape), an optical medium (for example, DVD), or a semiconductor medium (for example, a solid state disk (SolidStateDisk, SSD)) and the like.
PUM
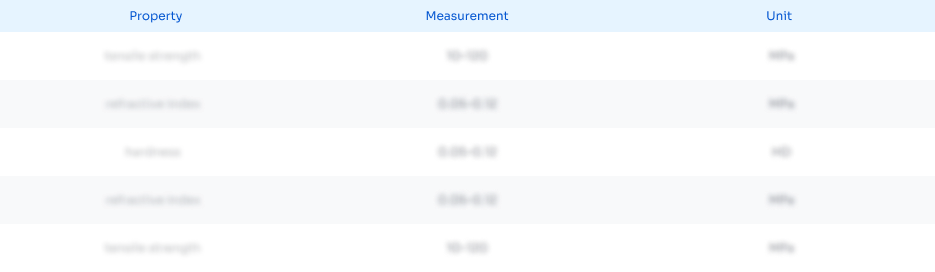
Abstract
Description
Claims
Application Information
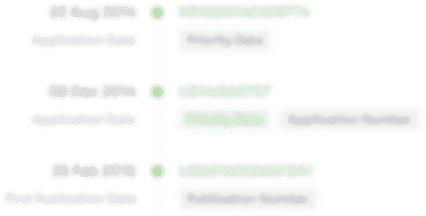
- R&D Engineer
- R&D Manager
- IP Professional
- Industry Leading Data Capabilities
- Powerful AI technology
- Patent DNA Extraction
Browse by: Latest US Patents, China's latest patents, Technical Efficacy Thesaurus, Application Domain, Technology Topic, Popular Technical Reports.
© 2024 PatSnap. All rights reserved.Legal|Privacy policy|Modern Slavery Act Transparency Statement|Sitemap|About US| Contact US: help@patsnap.com