Patient-level tumor intelligent diagnosis method based on full-view digital slicing
A technology of digital slicing and diagnostic methods, which is applied in the field of patient-level tumor intelligent diagnosis, can solve problems such as misdiagnosis and rapid diagnosis at the difficult case level, and achieve the effects of increasing the receptive field, reducing manual operations, and enhancing capabilities
- Summary
- Abstract
- Description
- Claims
- Application Information
AI Technical Summary
Problems solved by technology
Method used
Image
Examples
Embodiment 1
[0033] In this embodiment, intelligent staging diagnosis is performed on the full-field digital slices in the lung cancer database. The lung cancer database is a lung cancer database composed of 225 cases. There are 1071 full-field digital slices, of which 683 full-field digital slices are positive, and 388 The full-field digital slices were negative, that is, no tumor tissue was included. All full-field digital slices were cut into small pieces, a total of 161,973 pieces took up 92.2Gb of memory, and all full-field digital slices were extracted with thumbnails, a total of 1071 pieces took up 6.3Gb of memory.
[0034] The details of each step and the setting of model parameters are described in detail below.
[0035] Step 1, load case data: Traverse all files with the suffix .wsi format under the case path.
[0036] Use the os library in the python language to traverse the database of all cases to obtain the storage path of the cases. Use the sklearn library to divide 225 cas...
PUM
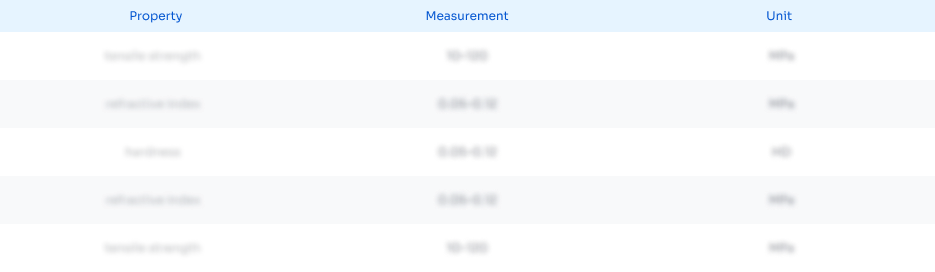
Abstract
Description
Claims
Application Information
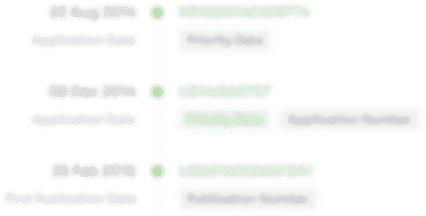
- R&D Engineer
- R&D Manager
- IP Professional
- Industry Leading Data Capabilities
- Powerful AI technology
- Patent DNA Extraction
Browse by: Latest US Patents, China's latest patents, Technical Efficacy Thesaurus, Application Domain, Technology Topic, Popular Technical Reports.
© 2024 PatSnap. All rights reserved.Legal|Privacy policy|Modern Slavery Act Transparency Statement|Sitemap|About US| Contact US: help@patsnap.com