Neural network training method and device and computer equipment
A neural network and training method technology, applied in the field of devices, computer equipment, and neural network training methods, can solve problems such as inability to utilize knowledge and affect students' network distillation learning efficiency, and achieve the effect of improving training efficiency.
- Summary
- Abstract
- Description
- Claims
- Application Information
AI Technical Summary
Problems solved by technology
Method used
Image
Examples
Embodiment 1
[0041] see figure 1 As shown, it is a flow chart of the neural network training method provided by the embodiment of the present disclosure, the method includes steps S102-S108, wherein:
[0042] S102: Obtain a sample image; input the sample image to the first neural network and a plurality of second neural networks for classification, obtain the output data of the first preset network layer of the first neural network and each of the second neural networks The output data of the second preset network layer of the neural network, wherein the second neural network is used for distillation training of the first neural network.
[0043] In the embodiment of the present disclosure, the first neural network may be understood as a student network to be trained, and the second neural network may be understood as a teacher network for training the student network to be trained. Compared with the second neural network, the first neural network has a simpler structure and fewer network...
Embodiment 2
[0073] On the basis of the technical solution described in the first embodiment above, in the embodiment of the present disclosure, as figure 2 As shown, the method also includes the following steps:
[0074] Step S201: Determine a second target loss value based on the category label information of the sample image and the category prediction result of the sample image by the first neural network.
[0075] Based on this, the above-mentioned step of iteratively adjusting the network parameters of the first neural network according to the first target loss value may further include the following step S202: according to the first target loss value and the second target loss value The determined joint loss value iteratively adjusts network parameters of the first neural network. Wherein, the joint loss value may be the sum or average value of the first target loss value and the second target loss value. It should be noted that, in the embodiment of the present disclosure, the e...
Embodiment 3
[0092] In the embodiment of the present disclosure, on the basis of the above-mentioned embodiment 1 and embodiment 2, as image 3 As shown, based on the preset objective function, the weight values respectively corresponding to the second neural networks are determined, including the following steps:
[0093] Step S301: Minimize the L2 norm on the objective function to obtain the optimal solution of the preset weight variable used to weight the gradient of the first loss value in the objective function, and convert the optimal A solution is determined as the weight value of the second neural network.
[0094] Specifically, in the embodiment of the present disclosure, the objective function may be solved and calculated by the L2 norm minimization solution method, and the obtained optimal solution of the preset weight variable is the weight value of the second neural network.
[0095] The formula of the above objective function can be expressed as: Among them, θ (τ) is th...
PUM
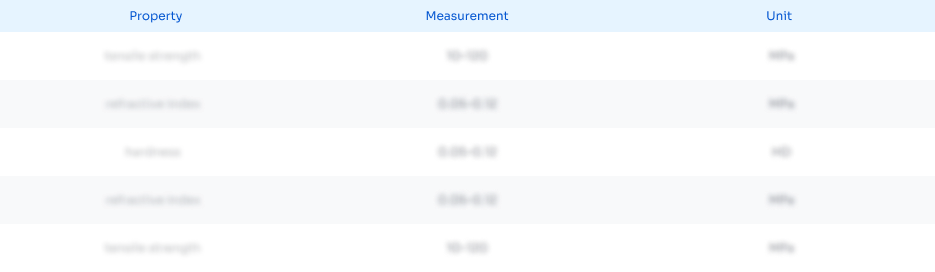
Abstract
Description
Claims
Application Information
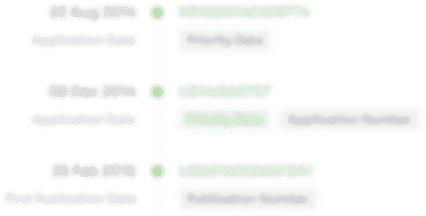
- Generate Ideas
- Intellectual Property
- Life Sciences
- Materials
- Tech Scout
- Unparalleled Data Quality
- Higher Quality Content
- 60% Fewer Hallucinations
Browse by: Latest US Patents, China's latest patents, Technical Efficacy Thesaurus, Application Domain, Technology Topic, Popular Technical Reports.
© 2025 PatSnap. All rights reserved.Legal|Privacy policy|Modern Slavery Act Transparency Statement|Sitemap|About US| Contact US: help@patsnap.com