Robot-oriented deep learning model segmentation method under cloud-edge-terminal framework
A deep learning and robotics technology, applied in manipulators, program-controlled manipulators, manufacturing tools, etc., can solve problems such as time-consuming, affecting the processing time of deep learning models, and not making full use of end-to-end computing power, to achieve the effect of improving inference speed.
- Summary
- Abstract
- Description
- Claims
- Application Information
AI Technical Summary
Problems solved by technology
Method used
Image
Examples
Embodiment Construction
[0025] In order to make the objectives, technical solutions and advantages of the present invention clearer, the present invention will be further described in detail in conjunction with the accompanying drawings and embodiments. It should be understood that the specific embodiments described here are only used to explain the present invention, rather than all Example. Based on the embodiments of the present invention, all other embodiments obtained by persons of ordinary skill in the art without making creative efforts are within the protection scope of the present invention.
[0026] Such as figure 1 As shown, the present invention provides a flow chart of a deep learning model segmentation method under a robot-oriented cloud-edge-end architecture, and the specific steps are as follows:
[0027] Step 1, in the robot cloud-edge-device architecture scenario, a monitoring server has a deep learning model, and models the deep learning model as a directed acyclic graph, wherein ...
PUM
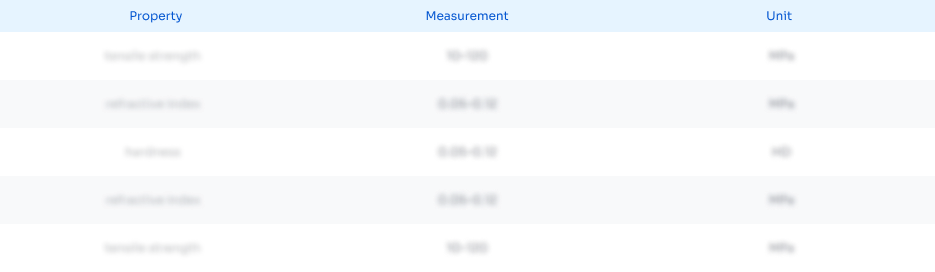
Abstract
Description
Claims
Application Information
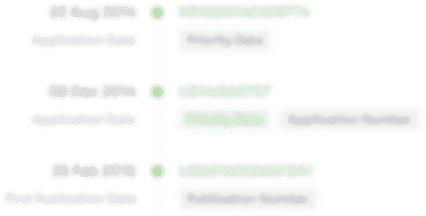
- R&D
- Intellectual Property
- Life Sciences
- Materials
- Tech Scout
- Unparalleled Data Quality
- Higher Quality Content
- 60% Fewer Hallucinations
Browse by: Latest US Patents, China's latest patents, Technical Efficacy Thesaurus, Application Domain, Technology Topic, Popular Technical Reports.
© 2025 PatSnap. All rights reserved.Legal|Privacy policy|Modern Slavery Act Transparency Statement|Sitemap|About US| Contact US: help@patsnap.com