Network coupling time sequence information flow prediction method based on causal logic and graph convolution feature extraction
A feature extraction and network coupling technology, applied in prediction, neural learning methods, biological neural network models, etc.
- Summary
- Abstract
- Description
- Claims
- Application Information
AI Technical Summary
Problems solved by technology
Method used
Image
Examples
Embodiment 1
[0058] Embodiment 1: see figure 1 — Figure 5 , a network coupling temporal information flow prediction method based on causal logic and graph convolution feature extraction, the method comprising the following steps:
[0059] S1, based on the time series data of N sampling nodes, use transfer entropy (Transferentropy) to establish a causal logic network G=(V,E);
[0060] Transfer entropy is a measure of the dependence between two random events X and Y in information theory, the expression is:
[0061]
[0062] where entropy H X =-∑ x p(x) log 2 p(x) measures the uncertainty of a discrete random variable X under the probability distribution p(x), is the k-order time-delayed subsequence of the known process X The conditional entropy when is the k-order time-delay subsequence of the known process X and the l-order time-delay subsequence of the process Y Conditional entropy at time. If TE Y→X >0, there is information flow between Y and X.
[0063] A causal log...
PUM
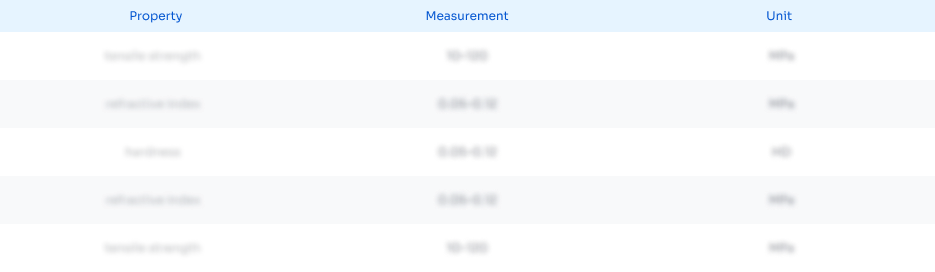
Abstract
Description
Claims
Application Information
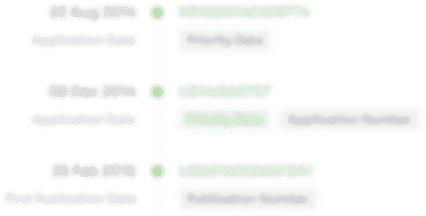
- R&D Engineer
- R&D Manager
- IP Professional
- Industry Leading Data Capabilities
- Powerful AI technology
- Patent DNA Extraction
Browse by: Latest US Patents, China's latest patents, Technical Efficacy Thesaurus, Application Domain, Technology Topic, Popular Technical Reports.
© 2024 PatSnap. All rights reserved.Legal|Privacy policy|Modern Slavery Act Transparency Statement|Sitemap|About US| Contact US: help@patsnap.com