Bearing fault mode diagnosis method and system for small sample data set
A technology of failure modes and diagnostic methods, applied in neural learning methods, electrical digital data processing, character and pattern recognition, etc., to avoid distribution inconsistencies and alleviate performance degradation.
- Summary
- Abstract
- Description
- Claims
- Application Information
AI Technical Summary
Problems solved by technology
Method used
Image
Examples
Embodiment
[0074] The present invention provides a bearing fault mode diagnosis method for small sample data sets, such as Figure 4 shown, including the following steps:
[0075] 1) The time-series signal of bearing vibration collected in this example has four different working conditions. Each working condition includes 10 bearing failure modes. In addition to normal, there are 9 failure types, including three different failures Location: inner race fault, ball fault and outer race fault, and each fault location has three different fault sizes.
[0076] 2) After continuous wavelet transform and image grayscale of various types of bearing signals, a characteristic map of bearing faults is formed, some of which are as follows: figure 1 As shown, it is finally stored in the database of the server.
[0077] Step 2) specifically includes:
[0078] Step 201: The vibration data collected by the acceleration sensor is a one-dimensional continuous time series signal, and the signal is prepro...
PUM
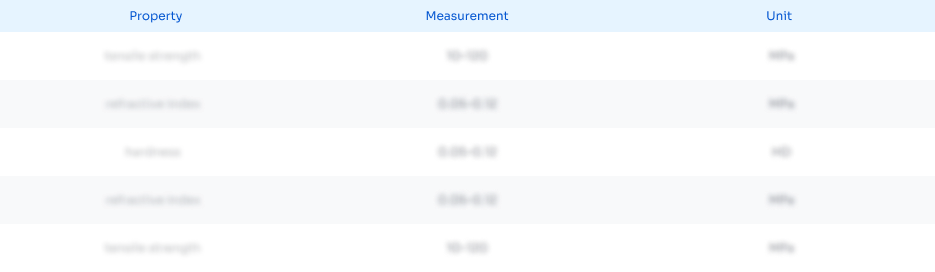
Abstract
Description
Claims
Application Information
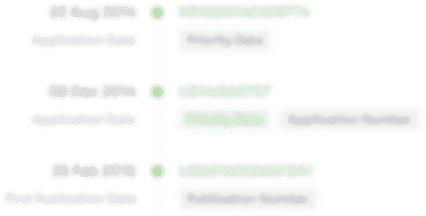
- R&D
- Intellectual Property
- Life Sciences
- Materials
- Tech Scout
- Unparalleled Data Quality
- Higher Quality Content
- 60% Fewer Hallucinations
Browse by: Latest US Patents, China's latest patents, Technical Efficacy Thesaurus, Application Domain, Technology Topic, Popular Technical Reports.
© 2025 PatSnap. All rights reserved.Legal|Privacy policy|Modern Slavery Act Transparency Statement|Sitemap|About US| Contact US: help@patsnap.com