STN-based autism brain magnetic resonance image visualization method
A technology of magnetic resonance images and nuclear magnetic resonance images, which is applied in the field of visualization of autistic brain magnetic resonance images based on STN, can solve the problems of lack of input image invariance and small transformation range
- Summary
- Abstract
- Description
- Claims
- Application Information
AI Technical Summary
Problems solved by technology
Method used
Image
Examples
Embodiment Construction
[0049] The present invention will be further described in detail below in conjunction with the accompanying drawings and specific embodiments.
[0050] An STN-based visualization method for magnetic resonance images of the autistic brain, the specific method is as follows:
[0051] 1) Collect brain MRI images of autistic patients as training samples;
[0052] 2) Use the training samples to train the STN model to obtain transformed images;
[0053] 21) Construct the STN model and initialize the network parameters randomly;
[0054] Construct the STN model, such as figure 1 , the STN model includes three parts: localization network (Localisation Network), grid generator (Grid Generator), and sampler (Samper). Among them: Localisation Network is a small convolutional neural network, and the input feature map U passes through multiple The convolutional layer and the pooling layer, and then through the fully connected and regression layers, generate the spatial transformation pa...
PUM
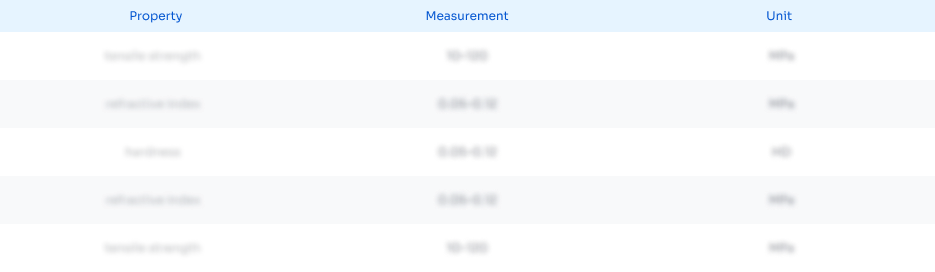
Abstract
Description
Claims
Application Information
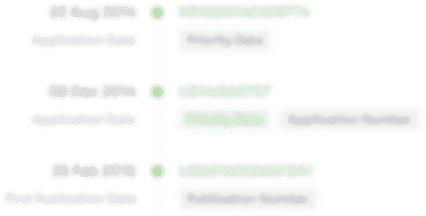
- R&D Engineer
- R&D Manager
- IP Professional
- Industry Leading Data Capabilities
- Powerful AI technology
- Patent DNA Extraction
Browse by: Latest US Patents, China's latest patents, Technical Efficacy Thesaurus, Application Domain, Technology Topic, Popular Technical Reports.
© 2024 PatSnap. All rights reserved.Legal|Privacy policy|Modern Slavery Act Transparency Statement|Sitemap|About US| Contact US: help@patsnap.com