Automatic feature construction method based on attention mechanism and reinforcement learning
A technology of reinforcement learning and construction methods, applied in machine learning, based on specific mathematical models, computer components, etc., can solve problems such as poor scalability, cumbersome, and difficult to generalize, and achieve efficient feature selection process and optimization iteration efficiency. Effect
- Summary
- Abstract
- Description
- Claims
- Application Information
AI Technical Summary
Problems solved by technology
Method used
Image
Examples
Embodiment 1
[0085] The recommendation system usually needs to meet the recommendation explanatory, that is, while providing the recommendation to the user, the reason for the recommendation is given. Therefore, the recommendation system data set is very suitable for verifying the interpretability of the model. The present invention uses the MovieLens-1M data set commonly used in related experiments in the recommendation field as an example to conduct experiments. The MovieLens-1M data set contains 1 million user rating data for 4000 movies from 6000 users. The detailed statistical information is shown in Table 1.
[0086] Table 1 Statistics of MovieLens-1M dataset
[0087]
[0088] The present invention automatically learns feature combinations based on the similarity of features in global data. Specifically, the present invention measures the correlations among feature fields according to their average attention scores in the whole data, which are obtained by the attention scores. T...
Embodiment 2
[0091] Compared with manual mining feature combination, the model of the present invention achieves finer-grained feature combination, and the process is completely transparent. image 3 Shows the correlations between different eigenvalues of the input features, which are obtained by attention scores. The color depth represents the attention score of the vertical axis element under the horizontal axis element, and the darker the color, the larger the value.
[0092] from image 3 It can be analyzed that the automatic feature generation method based on the self-attention mechanism proposed by the present invention can identify meaningful combination features ( i.e. solid line rectangle). This makes perfect sense, since young college girls are likely to prefer movies in the romance genre. Generally speaking, high-order feature combinations are difficult for manual methods, and it takes a lot of energy and experience to dig out high-order features. However, the feature inter...
Embodiment 3
[0094] Due to the characteristics of the small-sample learning method of SVM, four public supervised classification datasets with small sample size are selected for the experimental data. The statistics of these datasets are summarized in Table 1, which contain inconsistent number of instances, number of features, and class imbalance. All datasets are available in the OpenML repository (http: / / www.openml.org / home).
[0095] Table 1 Statistical information of the data sets in the comparison experiment of machine learning methods
[0096]
[0097] The model has only two hyperparameters: the maximum number of iterations is set to 10, and the embedding size is set to 3. All experiments take the average value after 5-fold cross-validation as the final result, and Table 2 shows the experimental results.
[0098] Table 2 Comparison of machine learning methods (based on SVM classifier)
[0099]
[0100] From Table 2, it can be seen that compared with the other three methods tha...
PUM
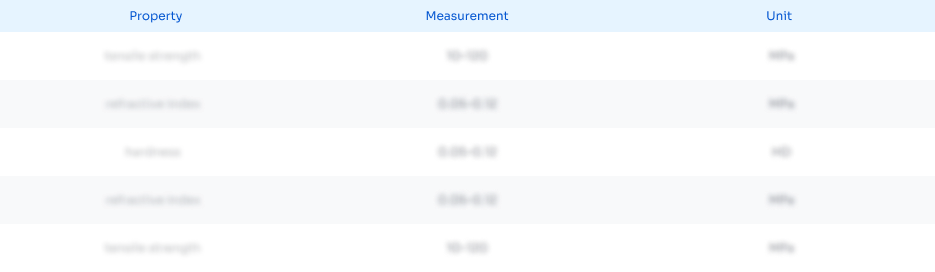
Abstract
Description
Claims
Application Information
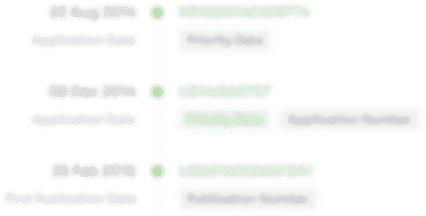
- R&D Engineer
- R&D Manager
- IP Professional
- Industry Leading Data Capabilities
- Powerful AI technology
- Patent DNA Extraction
Browse by: Latest US Patents, China's latest patents, Technical Efficacy Thesaurus, Application Domain, Technology Topic, Popular Technical Reports.
© 2024 PatSnap. All rights reserved.Legal|Privacy policy|Modern Slavery Act Transparency Statement|Sitemap|About US| Contact US: help@patsnap.com