Manifold optimization based method for non-smooth 3D image completion of tensor low-rank models
A three-dimensional image, non-smooth technology, applied in image enhancement, image data processing, instruments, etc., can solve the problems of poor image arrangement adaptability, lack of prior interpretation, limited application scenarios, etc., to achieve good interpretability and scope of application Increase, the effect of good complementary effect
- Summary
- Abstract
- Description
- Claims
- Application Information
AI Technical Summary
Problems solved by technology
Method used
Image
Examples
Embodiment Construction
[0070] Below in conjunction with the accompanying drawings, the present invention is further described by means of embodiments, but the scope of the present invention is not limited in any way.
[0071] The invention provides a non-smooth three-dimensional image completion method based on a manifold-optimized tensor low-rank model MOTQN, which utilizes manifold optimization to update a data-dependent orthogonal projection basis for efficiently performing low-rank non-smooth three-dimensional images. complete tasks, Figure 4 Shown is the specific implementation process of the method of the present invention to realize the non-smooth 3D image completion based on the manifold-optimized tensor low-rank model, including the following steps:
[0072] Step 1: Select limited 3D image observation samples Suppose it is the original non-smooth 3D image to be restored through a projection operator Obtained from the action of the indicator set Ω. The data set used in this example i...
PUM
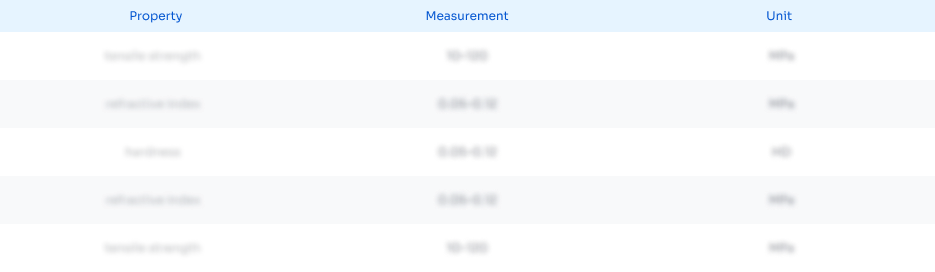
Abstract
Description
Claims
Application Information
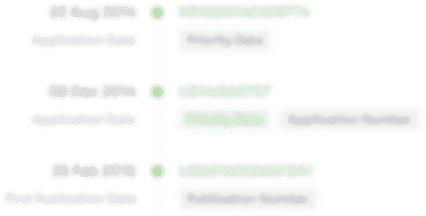
- R&D
- Intellectual Property
- Life Sciences
- Materials
- Tech Scout
- Unparalleled Data Quality
- Higher Quality Content
- 60% Fewer Hallucinations
Browse by: Latest US Patents, China's latest patents, Technical Efficacy Thesaurus, Application Domain, Technology Topic, Popular Technical Reports.
© 2025 PatSnap. All rights reserved.Legal|Privacy policy|Modern Slavery Act Transparency Statement|Sitemap|About US| Contact US: help@patsnap.com