Event time sequence relationship recognition method based on relation graph attention neural network
A time-series relationship, neural network technology, applied in neural learning methods, biological neural network models, neural architectures, etc., can solve the problems of omission and loss, and it is difficult to effectively process long-distance non-local semantic information.
- Summary
- Abstract
- Description
- Claims
- Application Information
AI Technical Summary
Problems solved by technology
Method used
Image
Examples
Embodiment Construction
[0035] In order for the skilled person to better understand the present invention, the present invention will be further explained below in conjunction with accompanying drawings and specific examples, and the specific details are as follows:
[0036] The present invention comprises the steps:
[0037] Step1: Timing diagram construction.
[0038] Firstly, semantic dependency analysis is performed on event sentence pairs to obtain two dependency trees. For each dependency tree, find the position of the trigger word, and use the trigger word as the starting point, recursively search its adjacent nodes until the adjacent node of p hops, and keep the searched nodes in this stage, where p is number of recursions.
[0039] In order to strengthen the semantic connection between event sentence pairs and the semantic representation between long-distance participles, some artificially constructed edges were added later. In order to simplify operations and improve computing power, the...
PUM
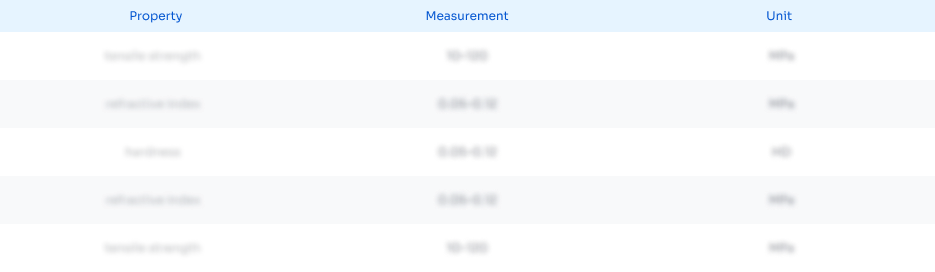
Abstract
Description
Claims
Application Information
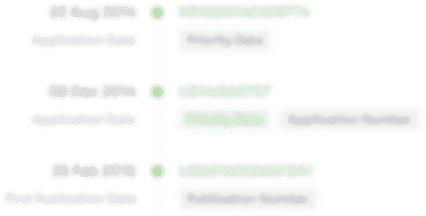
- R&D
- Intellectual Property
- Life Sciences
- Materials
- Tech Scout
- Unparalleled Data Quality
- Higher Quality Content
- 60% Fewer Hallucinations
Browse by: Latest US Patents, China's latest patents, Technical Efficacy Thesaurus, Application Domain, Technology Topic, Popular Technical Reports.
© 2025 PatSnap. All rights reserved.Legal|Privacy policy|Modern Slavery Act Transparency Statement|Sitemap|About US| Contact US: help@patsnap.com