Real-time anomaly detection method based on generative adversarial network
An anomaly detection and real-time detection technology, applied in biological neural network models, neural learning methods, structured data retrieval, etc., can solve problems such as algorithm bias, unsupervised methods are difficult to function, and normal data is mistakenly detected as abnormal data.
- Summary
- Abstract
- Description
- Claims
- Application Information
AI Technical Summary
Problems solved by technology
Method used
Image
Examples
Embodiment Construction
[0029] In order to make the purpose, content, and advantages of the present invention clearer, the specific implementation manners of the present invention will be further described in detail below in conjunction with the accompanying drawings and embodiments.
[0030] The present invention is divided into two parts: off-line training and real-time detection. The flow chart of the overall scheme is as follows: figure 1 shown. In the offline part, the batch of historical normal data collected from the production equipment is firstly input into the generative confrontation network model after data cleaning. The model independently generates abnormal data and conducts identification detection. After the training is completed, the model is deployed to the real-time detection environment.
[0031] The real-time detection part first collects real-time data from the production equipment, and stores the data in the historical database synchronously. After the real-time data is cleaned...
PUM
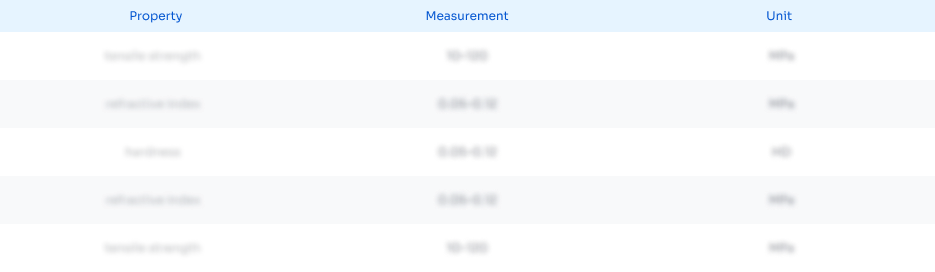
Abstract
Description
Claims
Application Information
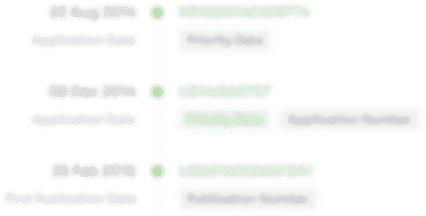
- R&D Engineer
- R&D Manager
- IP Professional
- Industry Leading Data Capabilities
- Powerful AI technology
- Patent DNA Extraction
Browse by: Latest US Patents, China's latest patents, Technical Efficacy Thesaurus, Application Domain, Technology Topic, Popular Technical Reports.
© 2024 PatSnap. All rights reserved.Legal|Privacy policy|Modern Slavery Act Transparency Statement|Sitemap|About US| Contact US: help@patsnap.com