SE-FPN-based target detection model training method and target detection method and device
A technology of SE-FPN and target detection, which is applied in the field of computer vision, can solve problems affecting detection accuracy, waste of resources, uneven distribution of targets, etc., and achieve the effect of enriching semantic information
- Summary
- Abstract
- Description
- Claims
- Application Information
AI Technical Summary
Problems solved by technology
Method used
Image
Examples
Embodiment 1
[0063] This example provides a SE-FPN-based target detection model training method, such as figure 1 As shown, the process includes the following steps:
[0064] S100: First randomly select four training pictures from the data set, scale them according to different scaling factors, and then splicing them into a new picture whose resolution is the input resolution set by the network model. The new picture includes multiple different sizes Target.
[0065] The target labels corresponding to the four pictures are scaled and spliced in the same way as the picture, so as to maintain the consistency between the new image and the target label.
[0066] When the target is multi-category, first count the number of samples of each category, calculate the probability of each sample being drawn according to the statistical results, generate the sampling probability of the data set, and draw samples according to the sampling probability each time.
[0067] S200: Allocate multiple obje...
Embodiment 2
[0089] This example provides a SE-FPN-based target detection model training device, such as Figure 7 As shown, it includes image preprocessing module, feature extraction module, target distribution module, sample selection module, and calculation module.
[0090] The image preprocessing module scales the multiple training pictures obtained from the data set according to different scaling factors, and stitches them into a new picture, and performs the same scaling and splicing processing on the target labels corresponding to the multiple training pictures, and the new The picture includes multiple objects of different sizes.
[0091] The target allocation module allocates multiple targets of different sizes to different pyramid feature layers of the SE-FPN target detection model according to a predetermined allocation strategy.
[0092] In each pyramid feature layer, the sample selection module finds the nearest m positions, respectively m positions of all anchors and groun...
Embodiment 3
[0095] This example provides a target detection method based on the SE-FPN target detection model, such as Figure 8 shown, including the following steps:
[0096] S100: Acquire an image to be detected.
[0097] S200: Input the image to be detected into the pre-trained SE-FPN target detection model; wherein, the SE-FPN target detection model is trained by using the SE-FPN-based target detection model training method in Embodiment 1.
[0098] S300: Detect the image to be detected by using the SE-FPN target detection model to obtain a target detection result; the target detection result includes position information of the target object in the image to be detected.
PUM
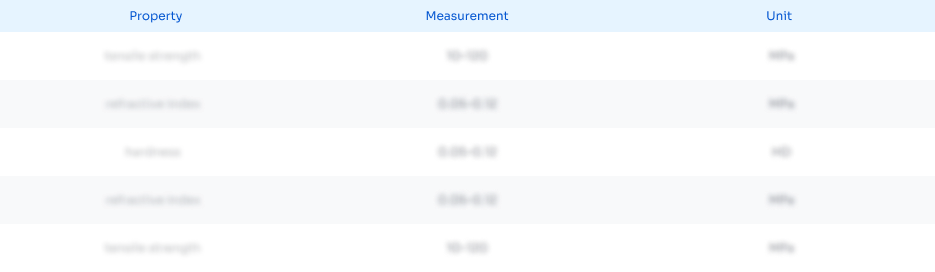
Abstract
Description
Claims
Application Information
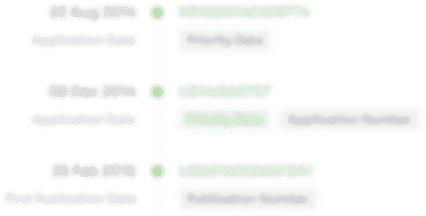
- R&D
- Intellectual Property
- Life Sciences
- Materials
- Tech Scout
- Unparalleled Data Quality
- Higher Quality Content
- 60% Fewer Hallucinations
Browse by: Latest US Patents, China's latest patents, Technical Efficacy Thesaurus, Application Domain, Technology Topic, Popular Technical Reports.
© 2025 PatSnap. All rights reserved.Legal|Privacy policy|Modern Slavery Act Transparency Statement|Sitemap|About US| Contact US: help@patsnap.com